16 Use of AI in Teaching Social Statistics or Data Analysis
Mudasir Mustafa
Abstract
In face-to-face classrooms, culturally and critically conscious teachers fight to mediate skills, content, and students’ backgrounds all within the bounds of a systematically flawed educational institution. For now, AIs that aid in writing bypass that kind of meaningful work as AIs reflect the corpus of published literature that overwhelmingly reflects historically problematic hierarchical biases.
Keywords: data analysis and AI utilization, statistics and AI accuracy, statistics and AI
My recent experience teaching an online course on Social Statistics revealed two significant aspects of Artificial Intelligence (AI) usage: (i) student over-reliance on AI for accuracy, and (ii) concerns about AI reinforcing racially-biased concepts in reference categories.
Firstly, a substantial number of students exhibit a steadfast belief in AI’s miraculous problem-solving capabilities, and they often adopt AI-generated solutions without validating their accuracy. For instance, in one of the given assignments in my class, I asked them to calculate the standard deviation and provide the solution with calculations. Several students provided solutions with calculations, but their answers were incorrect. It alerted me that students may be using AI to solve this assignment. As an experiment, I input the same dataset into a popular AI chatbot, witnessing its persistence in providing incorrect responses; then, I provided the accurate answer, and the chatbot apologized and gave the correct answer (Figure 1 or Figure 2, containing full chat). This experiment underscored the necessity of fostering student awareness regarding the limitations of AI.
Secondly, AI’s interpretation of data analysis tests can perpetuate racial bias, particularly when using majority groups as reference categories. AI algorithms, relying on historical datasets, may unconsciously replicate societal biases present in the data. While teaching the same Social Statistics course, I queried two popular AI chatbots about providing an example of logistic regression with code and interpretation by specifying race as an independent variable and HIV diagnosed as a dependent variable (I also repeated the query with HIV prevalence, HIV test uptake). Both AI- generated codes chose “White Americans” as the reference category, providing interpretations without contextual caution or elaborating on the reasons for selecting White Americans as a reference category. It interpreted the result, such as, “in this example, Black Americans are 2.5 times more likely to be diagnosed with HIV than White Americans.” Upon my next query, “why you choose the White Americans as a reference category,” one AI replied that it chose it randomly, while the other enlisted some reasons such as considering it as a largest group with having more power and privilege, as well as considering it as having lowest HIV prevalence group (Figure 2). Selecting a reference category as “random” without any theoretical grounds led to several concerns, especially for students who are in the initial stage of learning statistics. It may reinforce the idea that White Americans are the default group, and that other racial groups are somehow different or abnormal. It also perpetuates the stereotype that HIV is primarily a disease of Black Americans, while obscuring the fact that HIV affects people of all races and ethnicities. This may lead to inaccurate conclusions about the prevalence and risk factors for HIV infection.
However, these AI have the ability to customize answers to forthcoming questions as per your previous chat with the chatbot. My ongoing exploration into the intersection of AI and racial bias has revealed that AI systems can be sensitive to the potential generation of biased content. In a recent interaction with the AI chatbot, I received a warning about the sensitivity of using race as a variable; it commented, “research involving race and other sensitive variables with great care to avoid perpetuating stereotypes or inadvertently contributing to bias. When studying such topics, it’s crucial to consider ethical guidelines and consult with experts to ensure the research is conducted responsibly” (Figure 3). But upon experimenting with the same query on one of my friend’s chatbot who never entered any query related to racial disparities or HIV, she got the same above-mentioned biased content. Nevertheless, the customized answers illustrate the evolving landscape of AI awareness and the need for ongoing vigilance and critical reflection in its application.
Figure 1: Standard Deviation Error by ChatGPT
Figure 2: Addition Error by ChatGPT
Figure 3: Logistical Regression and Race Conversation with ChatGPT
Questions to Guide Reflection and Discussion
- Discuss the potential risks and inaccuracies when students rely solely on AI for solving statistical problems. How can educators ensure that students understand the underlying concepts?
- Reflect on the ethical implications of AI perpetuating racial biases in statistical analysis. How can this issue be addressed in the curriculum?
- How can instructors use AI as a tool to demonstrate real-world applications of statistics and data analysis, enhancing learning outcomes?
About the author
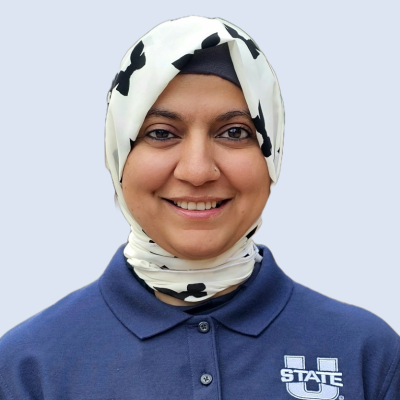
name: Mudasir Mustafa
institution: Utah State University
Mudasir Mustafa, a Ph.D. candidate in Sociology, focuses on understanding disparities in physical and mental well-being, maternal and child health, digital divide and role of technology, and the complex interplay of racial and sexual identities. Her dissertation explores the role of maternal adverse childhood experiences in non-live births, including miscarriages, stillbirths, and abortions.