Changes in Obstacles to Learning During the COVID-19 Pandemic for University Students and Recommended Solutions
Becky Williams, Ph.D. and Sunshine L. Brosi, Ph.D.
Abstract
The COVID-19 pandemic caused disruptions to student learning from K–12 to universities and continues to manifest negative effects on students. To better understand the challenges our students face and how those obstacles have changed since the COVID-19 pandemic began, we surveyed our undergraduate ecology students who ranked obstacles to learning they experience in technology, learning environment, and economic security. The majority of respondents report conditions have worsened since the onset of the pandemic. Surveys identified the largest challenges on average were being unfamiliar with technology, using a smartphone or tablet for coursework, balancing work and employment, and having trouble focusing and retaining information. A principal components analysis (PCA) identified that not having reliable internet, having children and other dependents in the home to care for, and not having a safe or private place to study were also common challenges. The PCA also indicated that food and housing insecurity outweighed job insecurity, which may indicate that our students are underemployed or poorly paid. Non-white females and first-generation college students face more obstacles than other groups. Surprisingly, the frequency of obstacles faced did not influence academic normalized learning gains (NLGs). Nor did students with a higher number of demographic markers that indicate historically underserved groups show lower NLGs. Mitigating obstacles to learning as the pandemic continues and new virus variants emerge will take a multi-faceted approach and understanding of each individual student’s challenges. Recommendations to mitigate the burden include students’ self-identified preferred solutions of having more flexible assignment dates, study zones with good wifi, and more asynchronous material. Access to updated computers would also be beneficial. Given that housing and food security scored highly in obstacles experienced, food banks on campus could assist in relieving some of this economic burden. Finally, strategies such as dividing online material into small chunks (< 15 minutes), followed by formative assessment opportunities for student metacognition (quizzes, reflections, discussions), then followed by synchronous sessions focused on active learning provided a strong support for learning for all students pre-pandemic and these strategies continue to transcend the pandemic and lessen its short- and long-term impacts.
Keywords: pandemic pedagogy, rural education, normalized learning gains
Introduction
During the 2020–2021 academic year, COVID-19 spread globally and students and teachers were faced with uncertainty, last-minute changes in curriculum, adaptation to new technologies, and a myriad of professional and personal changes. Educators hoped to mitigate obstacles to learning with resilient and flexible teaching strategies (e.g., Fabrey & Keith, 2021; Thurston, 2021). However, there were clear indications of added stresses on students and educators (e.g., Jakubowski et al., 2021; Sokal et al., 2020; Wang et al., 2020; Yanghi, 2022). The pedagogical literature focused on online learning moved front and center while educators sought to identify obstacles students face and solutions to challenges presented by the pandemic (see below). Social media sites such as Pandemic Pedagogy emerged to facilitate communication between educators, demonstrating the great need to share knowledge quickly (Schwartzman, 2020). Here we present a survey tool we developed to identify the common challenges students face, assess changes in challenges our students faced because of the pandemic, assess how these challenges affect student learning, and rank solutions to those challenges. To this end, we provide a quantitative analysis of the data self-reported by students during the first year of the pandemic. Finally, few studies attempt to assess the effect of obstacles to learning on student learning gains during the pandemic, and we investigate that relationship here.
When our data collection began in Fall 2020, little was known of pandemic-specific impacts on students. Since our study began, a plethora of studies have appeared, both predictive (based on prior knowledge of how students learn) as well as quantitative studies that tested these predictions. Negative effects of the pandemic on students include, but are not limited to, lack of access to technology and wifi needed for online learning, increasing caregiving responsibilities, loss of economic stability, and degrading mental health (e.g., Al-Mawee et al., 2021; Brodeur et al., 2021; Gonzales et al., 2020; Morgan, 2020; Mustafa, 2020; Wang et al., 2020; Warren & Bordoloi, 2020; Yanghi, 2022). These effects are magnified for people of color, sex and gender minorities, females, and first-generation college students (e.g., Gilbert et al., 2021; Kimble-Hill et al., 2020; Moore et al., 2021; Reyes, 2020; Soria et al., 2020; Wang et al., 2020; Warren & Bordoloi, 2020). Finally, the pandemic is not over and continues to negatively impact student learning and students in terms of mental health and the long-term consequences of learning losses (e.g., Donnelly & Patrinos, 2021; Yahgi, 2022).
Fortuitously, much pedagogical research describing effective online instruction was available pre-pandemic to inform instructors forced into new formats for their courses. Just a few examples of effective techniques we have used in our courses include: incorporating active learning (e.g., Freeman et al., 2014; Handelsman et al., 2004; 2007; Preszler et al., 2007; Tanner, 2009; Wood, 2009), flipped classrooms (e.g., Brewer & Movahedazarhouligh, 2018), interspersing lecture tidbits (< 15 min) with formative assessment to support student metacognition (Broadbent & Poon, 2015; Dirks et al., 2014; Hake 1998ab; Handelsman et al., 2004; 2007; Larsen et al., 2009; Ozan and Ozarslan, 2016; Roediger & Karpicke, 2006; Roediger et al., 2011), regular but flexible deadlines to prevent students from falling behind with online material (Ariely & Wertenbroch, 2002; Guàrdia et al., 2013; Michinov et al., 2011), a mix of synchronous and asynchronous material and interaction (Johnson, 2006; Schwartzman, 2020; Yamagata-Lynch, 2014;), and flexible and resilient pedagogy (e.g., Fabrey & Keith, 2021; Thurston et al., 2021). The negative effects of the ongoing pandemic continue to vex students, and learning losses already experienced can translate to long-term lost earning potential and declining mental health (e.g., Currie & Thomas, 2001; Donnelly & Patrinos, 2021; Yaghi, 2022). Challenges to students persist, e.g., increasing cost of education, decreasing earning potential, and increasing caregiving responsibilities, among others. Whether or not students face a global crisis such as the pandemic or their own personal crises, teaching strategies such as those above should be embraced moving forward and regardless of the current pandemic trajectory.
Ladson-Billings (2021) notes that the pandemic highlights weaknesses in our education system and rather than return to “normal,” we can use this opportunity to improve our teaching in any situation. In this way, we can shed “normal” policies and teaching strategies that are disadvantageous to marginalized groups, such as Black students (Ladson-Billings, 2021; Reyes, 2020; Strayhorn & DeVita, 2010), and better help all students. As faculty members in a university system that includes campuses around Utah state, our students often represent a mix of multiple marginalized identities; they are often geographically isolated in rural settings, place-bound, first-generation college students, parents, and working students (statistics collected each year by each statewide campus ). The COVID-19 pandemic continues to have disproportionate impacts on marginalized communities and has increased challenges for all students (e.g., Kimble-Hill et al., 2020; Moore et al., 2021; Reyes, 2020; Soria et al., 2020). Given our statewide students’ demographics, the potential negative impact of the pandemic could be exacerbated. The goal of our research was to first, determine the students’ challenges and how these challenges have changed during the pandemic; second, assess how these challenges affect students’ individualized learning gains in our courses; and third, to discover students’ priorities for solutions to alleviate these challenges. Finally, we note that students’ self-identified strategies to mitigate pandemic challenges can be employed to mitigate any challenges and can help all students, regardless of current events.
Methods
Our Classrooms
The institutional review board of Utah State University (USU) approved the procedures of this study (IRB #11402). We investigated a total of three courses taught by two different instructors, but with highly similar teaching methods. Each course consisted of a structured online environment with small lecture tidbits interspersed with opportunities for students to check their understanding (quizzes, discussions, reflections) that prepared students for synchronous meetings (twice weekly) focusing on active learning. Course 1: In the fall 2020 semester BIOL 2220/WATS2220: General Ecology was taught using interactive video conference (IVC) with a ZoomTM bridge and originating from the USU campus in Uintah Basin (Vernal, Utah) (enrollment = 5 students, 100% statewide campuses). Although an in-person option was available in the Vernal classroom for one student, after the first month, this student also chose to interact via ZoomTM similarly to the other students. Course 2: In the fall 2020 semester WILD 2200: Ecology of Our Changing World (Breadth Life Sciences) was taught using web-broadcast (ZoomTM) originating from the USU Eastern campus (Price, Utah) (enrollment 39 students; 46% statewide campuses; 54% Logan main campus). Course 3: The spring 2021 semester BIOL 2220/WATS 2220: General Ecology originated from USU Eastern (enrollment = 22 students; 27% statewide campuses; 73% at the Logan main campus). This section was taught using the Interactive Videoconferencing (IVC) system with the Zoom™ bridge. The course originated from an IVC classroom and a maximum of four students attended in-person, although this number varied throughout the semester. The same textbook and general course schedule was used in all of the courses. Because the course format and content were so similar and one instructor taught only one course with a small sample size (BLW; four respondents), all three courses were pooled for analysis.
Survey Deployment
We conducted three surveys: Survey 1. the pre-course assessment of ecology knowledge, Survey 2: the post-course assessment of ecology knowledge (Smith et al., 2019; Summers et al., 2018), and Survey 3: a survey of obstacles the students were facing, how these obstacles have changed during the pandemic, and preferred solutions to these challenges. The latter survey was generated by us, but also included questions from the US Department of Agriculture (2012) US Adult Food Security Survey Module. Each survey started with a video introduction by the coauthor that was not the course instructor and included standard informed consent language including that participants were required to be at least 18 years of age, participation was voluntary, and responses anonymous. The incentive for participation was extra-credit points for each survey they completed or submission of an alternative non-survey assignment for the same amount of extra credit. The alternative assignment to the obstacles survey was a reading assignment about the effects of the pandemic on learning with follow up questions, which required a similar time investment to the obstacles survey.
We created our anonymous surveys in Qualtrics with a link to a separate survey at the end of either the alternative assignment or survey submission to record participation and receive extra credit. The pre-survey of learning gains was available to students the first week of class and the post-survey was available the two weeks before final exams. Survey 3: The obstacles survey was available mid-semester (October 16–23 and February 12–19). To match the surveys, calculate normalized learning gains (NLGs), and associate NLGs with obstacles, students entered a unique identifier or self-generated identification codes (SGICs) that allowed them to remain anonymous. Our unique identifier was (1) the first 2 digits of your birth month [e.g., February (02)], (2) the first two letters of your high school mascot [e.g., Pirate (pi)], (3) your birth order [e.g., 1 = first born or only child, 2 = second born, etc.], and (4) first two letters of the town where you were born [e.g., Logan (lo)]. Here is an example: John Jacob Smith, born in February, whose mascot was a Pirate, who is the youngest of three children, and who was born in Logan would have the code 02pi3lo. This allowed us to connect all three surveys while students remained anonymous to us.
Obstacles Survey Content
Demographic information was requested, including age, education level of parents, ethnicity, gender, sexual orientation, and primary language in the childhood home. For the obstacles survey, 7–8 questions were asked under each of the three categories of Technology, Learning Environment, and Economic Security (Employment, Housing, and Food Security). Each of the three sections of questioning had specific, Likert-scale questions, with values of “Often,” “Sometimes,” or “Never.” These items were designed to be balanced between negatively and positively structured questions in an attempt to avoid presenting students with only negative statements that might bias our results and student outcomes by increasing anxiety. A follow-up question asked students to rank whether the condition they were experiencing was “Worse,” “Similar,” or “Better” since the COVID-19 pandemic began. We also asked students to volunteer additional challenges we did not cover. Finally, we asked students to rank potential solutions to the challenges they were facing.
Data Analysis
Coding
Surveys were coded and qualitative responses were reduced to binary numbers. Coding was done using reverse coding as needed; for example, negatively worded questions, such as “My internet has a data limit, which restricts my access to online content,” were coded with “Often” as a 3 (highest level of obstacle), “Sometimes” as a 2, and “Never” as a 1 (lowest level of obstacle). The positively worded question, “The internet at my house is very reliable,” was reverse-coded with “Often” as a 1 (reduced level of obstacle), “Sometimes” as a 2, and Never as a 3 (highest level of obstacle). The same process was used for potential solutions ranking by students. A value of “three” was assigned to “High Priority,” followed by “two” for “Medium Priority,” and then “one” for “Low Priority.” In addition, true/false and yes/no questions were coded as 1 or 2 with the higher number indicating an increased obstacle. Demographic factors were also summed with each factor shown to be associated with risk of a student being underserved or discriminated against in the literature receiving a score of 1. These factors included: non-traditional (> 25 years of age), first-generation college student, non-white, female, non-heterosexual, a language other than English spoken in the childhood home, and whether a student felt part of a stereotyped group. Summing demographic scores that potentially increased the risk of a student experiencing a more difficult educational environment resulted in higher scores for individuals possessing more demographic identities; this intersectionality of identities is important because multiple challenges can negatively and synergistically affect students (Núñez, 2014; Ro & Loya, 2015; Kramarczuk et. al. 2021).
Normalized Learning Gains
We calculated normalized learning gains (NLGs; Weber, 2009) for results from pre- and post-assessment as follows:
NLG = (learning gains)/(possible learning gains) = (post score – pre score)/(100% – pre score)
This allowed us to remove the effect of prior ecology knowledge on final scores and thus provided a more direct assessment of progress made during the course. Some surveys were removed from analysis due to suspect quality. For example, one student took 16 minutes on the pre-assessment and then under 3 minutes on the post-assessment. When students spent less than 15% of the time on the test than the original attempt, or took under three minutes to complete the post-assessment, those scores were removed from analysis.
Analyses
We summarized survey data by averaging coded scores for each potential obstacle. Obstacle scores for each student were also summed across each category of obstacle (Technology, Learning Environment, and Economic Security) and summed across all three categories. At-risk demographic factors were also summed. Graphing was completed in Excel, SigmaPlot Version 14.0 (Systat Software, San Jose, CA), or JMP® 9.0.2 (SAS Institute, Inc.).
Because we used count data that did not always conform to a normal distribution, we used a Generalized Linear Model with a Poisson Distribution and Log Link (e.g., Breslow, 1996) to assess the relationship between normalized learning gains (NLGs) and total obstacle score as well as demographic score. A principal components analysis (PCA; Abdi & Williams, 2010; Jolliffe, 2005) was used to reduce our multidimensional dataset (in this case multiple questions for each student) to vectors (principle components; PCs) that encompass the variation in fewer dimensions and quantifies which data (questions in our case) most heavily influence each vector (PC). Hence, we performed a PCA on each category of obstacle (Technology, Learning Environment, and Economic Security). This allows us to ascertain which questions are most important to capturing the variation in student responses. Finally, we used a Least Squares Regression to assess the relationship between NLGs and the first two PCs for each of the three categories of obstacles. This allowed us to ascertain whether certain challenges were more influential on NLGs. Analyses were performed with JMP® 9.0.2 (SAS Institute, Inc.).
After visualizing the data, we assessed a few select hypotheses. Mann-Whitney U Tests (McKnight & Najab, 2010) were used to evaluate whether non-white females faced more obstacles than non-white males or white females, whether first-generation college students and faced more obstacles, and whether NLGs varied between any of these comparisons.
Results
Response Rate and Demographics
We discarded incomplete surveys and surveys lacking unique identifiers. Survey response rate for all three courses was 72.7% (48 of 66 students) completing both the pre-course and post-course assessments, and 81.8% (54 of 66 students) completing the obstacles survey (Table 1). The demographics of our students were 10.9% non-traditional student age (over age 25), 38.2% first-generation college students, 76.4% white, 60% female, 83.0% straight (heterosexual), and 6.4% were raised with a language besides English in their childhood home. It is important to note that even though the students may have been under age 25, they may already be married or parents; this question was not asked in the survey. Challenges associated with child-rearing and care-giving were captured in the obstacles survey.
Course Name | Semester | Originating Campus | Number Enrolled | Survey Response Rate (%) | |
---|---|---|---|---|---|
Pre- and Post-Course | Obstacles | ||||
BIOL 2220 | Fall 2020 | Uintah Basin | 5 | 5 of 5, 100% | 4 of 5, 80.0% |
WILD 2200 | Fall 2020 | Eastern | 39 | 30 of 39, 76.9% | 32 of 39, 82.1% |
BIOL 2220 | Spring 2021 | Eastern | 22 | 13 of 22, 59.1% | 18 of 22, 81.8% |
Total | 66 | 48 of 66, 72.7% | 54 of 66, 81.8% |
Obstacles
A total of 54 respondents completed the obstacles survey. The average student spent about eight and a half minutes on the obstacles survey. Students experienced more obstacles in all categories overall since the pandemic began (Table 2). Changes in these circumstances since the pandemic were ranked in terms of where they were “Worse,” “Similar,” or “Better” (Figure 1). The highest values, indicating the greatest number of students who perceived their situation was “Worse” were in the areas of “I have trouble focusing and retaining information in my courses” (2.43) and “The internet at my house is very reliable” (2.33; Tables 3–5). Other obstacles that worsened include “I have a job or jobs that I work in addition to going to school during the semester” (2.19) and “I can easily watch videos on the internet at my house” (2.15; Tables 3–5).
Category | Average Score for Obstacles Currently Experienced | Range | Change Since Covid-19 | Range |
---|---|---|---|---|
Living Environment | 11.43 | 9–18 | 14.59 | 10–20 |
Economic Security | 10.50 | 7–16 | 12.37 | 8–17 |
Technology | 10.43 | 7–18 | 14.42 | 11–21 |
All Categories Combined | 27.69 | 16–45 | 44.38 | 31–55 |
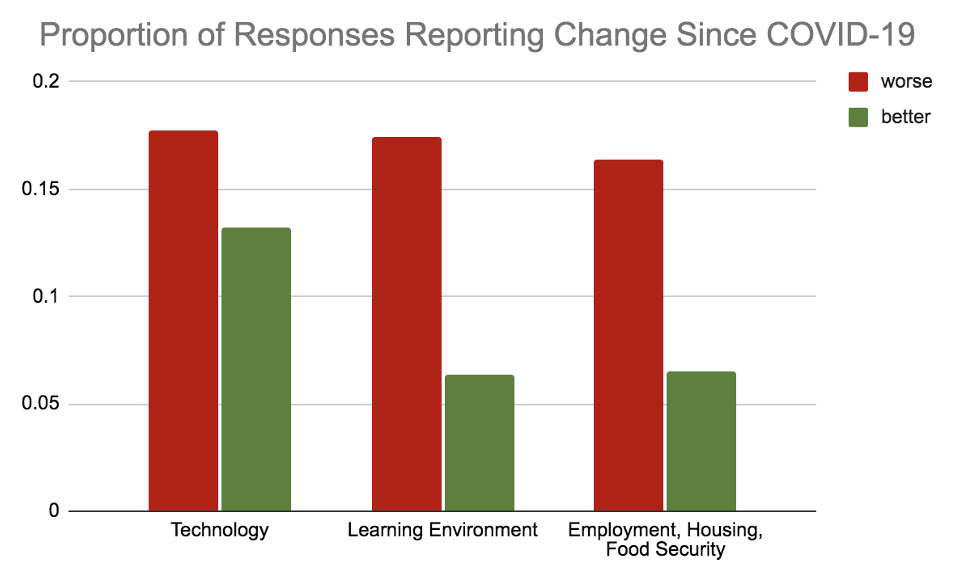
Higher scores indicate a greater preponderance of obstacles for those students. When asked about the students’ current obstacles the highest scores, e.g. largest percentage of “Often” facing the challenge, were for: “I have a job or jobs that I work in addition to going to school during the semester” (47.27%; 2.19) followed by “I have trouble focusing and retaining information in my courses” (32.73%; score 2.26). This represents one half and one third of all students reporting struggling with these challenges “Often.” Two technology issues also had high values, including “Technology and remote learning are familiar and comfortable for me” (12.72%; score 1.78) and “I use a smartphone or tablet to access course content, which can make viewing course content more difficult” (5.45%; score 1.66). Remember, positive statements were reverse-coded such that higher values indicate a larger magnitude of the obstacle; i.e. a high score on “Technology and remote learning are familiar and comfortable for me” indicated that many students found this not to be true and to represent a challenge. Overall, students faced the greatest number of challenges in the category of Learning Environment, followed by Economic Security, then Technology (Table 2). Detailed information for all three categories including current obstacles and changes since COVID-19 are summarized in Tables 3–5.
Technology | |||
---|---|---|---|
Question | Current Situation Average | Change Since Covid-19 | Percent of students experiencing obstacles in this area often |
Technology and remote learning are familiar and comfortable for me | 1.78 | 1.78 | 12.72% |
I use a smartphone or tablet to access course content, which can make viewing course content more difficult | 1.66 | 2.06 | 5.45% |
The internet at my house is very reliable | 1.49 | 2.33 | 5.45% |
My internet has a data limit, which restricts my access to online content | 1.46 | 2.06 | 14.55% |
The computer that I use for my education is older, unreliable, or inhibits me from accessing course content | 1.43 | 1.93 | 9.09% |
I can easily watch videos on the internet at my house | 1.40 | 2.15 | 1.82% |
I use a hotspot on my phone to access the internet | 1.41 | 2.13 | 5.45% |
Learning Environment | |||
---|---|---|---|
Question | Current Situation Average | Change Since Covid-19 | Percent of students experiencing obstacles in this area often |
I have trouble focusing and retaining information in my courses | 2.26 | 2.46 | 32.73% |
I have a door that I can close and room to myself to work on classes | 1.44 | 2.13 | 9.09% |
There are children in my home (my own kids, younger siblings, nieces/nephews, etc.) that I provide childcare for | 1.43 | 2.00 | 10.90% |
I have a washer and dryer in my home and I don’t have to worry about using a laundromat | 1.41 | 1.98 | 10.90% |
I am one of the primary people responsible for homeschooling or remote learning of young children in my home | 1.20 | 2.02 | 5.45% |
I feel safe at home to study for my courses | 1.20 | 2.00 | 3.63% |
I am a caretaker of an elderly or disabled person in my home | 1.15 | 2.08 | 3.63% |
Economic (Employment, Housing, and Food) Security | |||
---|---|---|---|
Question | Current Situation Average | Change Since Covid-19 | Percent of students experiencing obstacles in this area often |
I have a job or jobs that I work in addition to going to school during the semester | 2.19 | 2.19 | 47.27% |
I have reliable and consistent transportation to school and work | 1.39 | 1.94 | 9.09% |
The hours I work at my place of employment were reduced against my wishes | 1.36 | 2.08 | 9.09% |
Distance from campus | 1.33 | 2.00 | 16.36% |
In my house, we worried whether our food would run out before we got money to buy more | 1.32 | 2.11 | 7.27% |
I worried that I may get evicted from my house at any point in the past year | 1.19 | 2.09 | 1.81% |
In my house the food we bought just didn’t last, and we didn’t have money to get more | 1.15 | 2.00 | 1.81% |
In the category of Technology, the first two principal components (PCs) explained 52.5% of the variation in responses. The first PC (35.3%) for Technology obstacles was weighted on whether the internet was reliable, whether videos were easy to view, and whether a phone was used as an internet hotspot while the second PC (17.2%) was weighted on whether students had an old computer and whether they were familiar with distance technology. In the category of Learning Environment, the first two principal components (PCs) explained 46.7% of the variation in responses. The first PC (28.6%) for Learning Environment obstacles was weighted on whether there were children in the home, if the home felt safe, if other dependents were being cared for, and if students had a private room to study while the second PC (18.1%) was weighted on if students had trouble focusing, whether there were children to homeschool, and if the phone was a primary means of viewing course content. In the category of Economic Security, the first two principal components (PCs) explained 49.5% of the variation in responses. The first PC (29.8%) for Economic Security obstacles was weighted on worry about low food, worry about being evicted, and students actually running out of food while the second PC (19.7%) was weighted on job security and whether hours had been reduced at work.
Students were also asked to describe additional obstacles not addressed in the survey. A total of 33% of respondents supplied additional obstacles. These included extracurricular sports, stress, and additional challenges:
- Lack of motivation
- General stress and worry over the state of the nation
- Juggling time between, work, school, and family responsibilities
- I often find it difficult to maintain the motivation to finish my assignments. Most of the time I submit assignments only because I need to get it done rather than actually retaining the information and learning
- I learn better in person or through hands-on experience
- Noisy disturbances created by roommates and browsing the internet mid-session
- Basketball
- Staying on a computer all day gives me migraines
- Privacy to test and study when living in shared rooms, and communal rooms being under COVID-restrictions
- Online classes are not equal to in-person classes
- Not being able to meet with teachers face to face
- Death of a loved one, addiction, increased anxiety and depression due to social isolation
- Mental health
- It’s a lot harder to be motivated with online classes than with in-person classes
- Time for classes and safe place to study
- Not being able to meet new people or expand my social group. Also, most classes don’t have group work, so I feel like I have to struggle on my own because I don’t know anyone else in the class
- With some classes, the homework has been so overwhelming at times that I have very little to no time to de-stress, which also makes textbook reading all but impossible. I also have a child that has been experiencing some health issues for the last few months
Relationships Between Obstacles and Learning Gains
Of those that completed the obstacles survey, 47 completed both the pre- and post-course ecology assessments, allowing us to calculate normalized learning gains (NLGs). The pre-course assessments on average took students about 32 minutes to answer a series of 35 questions. The post-course assessments took an average of 21 minutes. There was no relationship between NLGs and the total summed score of obstacles from the three categories of challenges (Technology, Learning Environment, and Economic Security; χ2 = 0.1692; p = 0.6808). There was also no relationship between NLGs and demographic score (χ2 = 0.0092; p = 0.9238) and there was no interaction between obstacles and demographic factors (χ2 = 0.0211; p = 0.8843).
In order to determine if a subset of important questions (as identified by PCA) influenced NLGs disproportionately, we also regressed NLGs on the first two PCs for each of our three categories using a Least Squares Regression. There was no relationship between NLGs and each of the first two PCs for the three categories (R2 = 0.0640; F = 0.4556; p = 0.83465). A graph of NLGs vs. total score of obstacles across all three categories and using symbols to depict demographic factors shows that there is wide variation in NLGs across all levels of obstacle score and by demographic factors (Figure 2). Visualizing this data prompted us to test select hypotheses. Non-white female students experience more obstacles (x̅ = 39.20) than non-white male students (x̅ = 27.25; Figure 2A; Z = 2.3771; df =4,3; p = 0.0087) and white females (x̅ = 30.57; Z = 2.2803; df = 4,13; p = 0.0113). First-generation college students experience more obstacles (x̅ = 34.71) than students with parents that completed college (x̅ = 29.93; Figure 2B; Z = 2.3225; df = 29,16; p = 0.0101). There was no difference in NLGs for any of these comparisons.
Potential Solutions
In addition to assessing obstacles, students were to rank as “High”, “Medium,” or “Low” priority a wide-range of possible solutions to these problems (Table 6). Students prioritized “more flexible assignment deadlines” (2.41), followed by “individual study zones on campus for quiet and fast wifi” (1.98) and “more asynchronous material” (1.89). Over 50% of students prioritized “more flexible assignment deadlines” as a high priority (Table 6). Peer assistance groups in class, more time with the instructor, and food banks on campus ranked higher than more office hours and study groups outside of class, followed by laundry services and lactation rooms on campus.
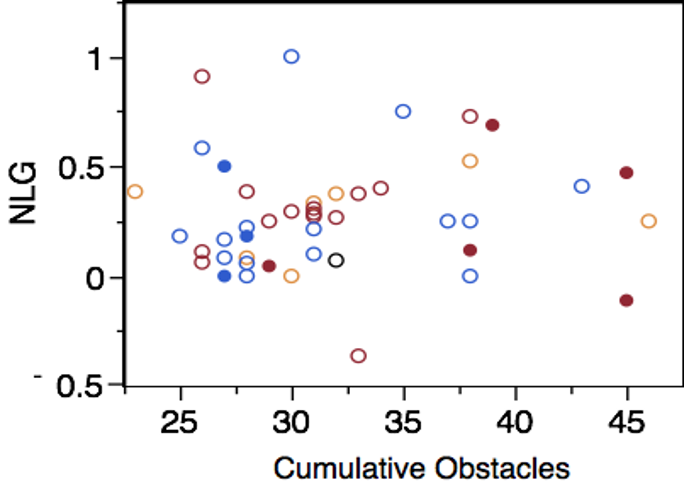
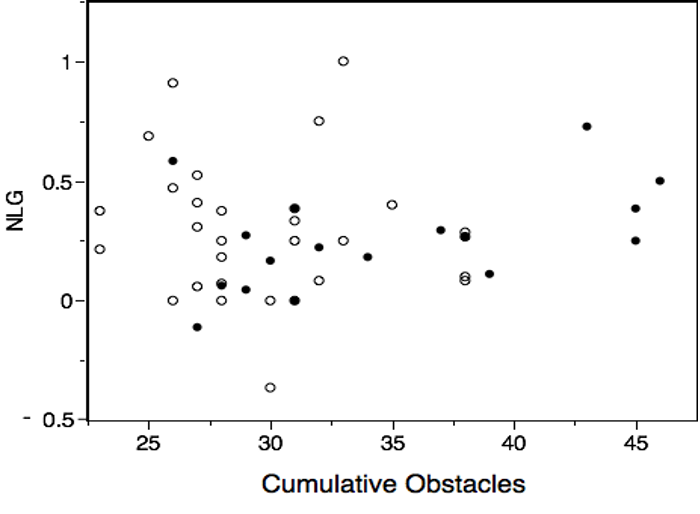
Solutions | ||
---|---|---|
Question | Average | Percent of students prioritizing this solution |
more flexible assignment deadlines | 2.41 | 50.90% |
individual study zones on campus for quiet and fast wifi | 1.98 | 36.36% |
more asynchronous material (on-your-own-schedule online material) | 1.89 | 18.18% |
creating peer assistance groups in class so you are not “invisible” in large enrollment classes | 1.83 | 20.00% |
additional personal meeting time with instructor | 1.80 | 12.72% |
food banks on campus | 1.74 | 20.00% |
additional office hours offered by the instructor | 1.72 | 16.36% |
facilitating the creation of study groups | 1.72 | 12.70% |
laundry services on campus | 1.61 | 18.18% |
lactation rooms on campus | 1.41 | 7.27% |
Discussion
Challenges Identified and Effect on Learning Gains
These surveys highlight that students at USU face significant obstacles to learning, and the COVID-19 pandemic increased the frequency of these hardships. Nearly half the students were working in addition to attending school. Many of our students face food or housing insecurity “Sometimes” and these obstacles are ranked above job security, which might suggest our students are underemployed or poorly paid. Though part-time employment is sometimes correlated to increased academic performance, as hours increase there is generally a detrimental impact on student success (Geel & Backes-Gellner, 2012). Students have increasing trouble focusing and retaining information while faced with multiple obstacles. A common problem pre-pandemic (e.g., Lang, 2020), trouble focusing was reported by a third of our students and scored the highest in magnitude of obstacle with respect to students’ Learning Environment. Learning technology tools for distance education and having equipment (computers rather than tablets/phones) and reliable internet are also significant obstacles for our students. While a global pandemic represents a large disturbance to all students, other disruptive events occur at smaller scales (e.g., individual events such as health issues or death of a loved one, etc.), and these disruptions can have similar effects on individual students; hence what we learned here can be used to improve student learning regardless of the current state of the COVID-19 pandemic. Overall, we identified and confirmed with data the role of many of the barriers others have suggested were amplified by the pandemic. Obstacles included lack of access to tech and wifi, employment obligations, caregiver responsibilities, and economic instability –– all of which are magnified for already marginalized groups (e.g., Brodeur et al., 2021; Kimble-Hill et al., 2020; Moore et al., 2021; Reyes, 2020; Schwartzman, 2020; Jacobs & King, 2002).
Non-white females experienced the highest score (combined number and magnitude) of challenges, while first-generation college students were close behind and also experience more challenges than other demographic groups (Figure 2AB). This highlights that intersectionality (the confluence of multiple identities), is an important consideration in evaluating obstacles faced by students (Núñez, 2014; Ro & Loya, 2015; Kramarczuk et. al. 2021). Unfortunately, our sample size and less demographically diverse population did not allow us to examine effects on groups holding multiple identities as in-depth as we would have liked. This lack of power may explain why we did not see an obvious effect of demographic factors on normalized learning gains (NLGs).
Despite a high response rate and relatively robust sample size, we found no relationship between obstacle score for individual students and Normalized Learning Gains (NLGs). We found high variance in NLGs across demographic groups and that may have obscured any relationship (Figure 2AB). This initial surprising result suggests that we did not capture the complexity of factors that affect NLGs. One explanation could be that assessments of NLGs were confounded by lack of investment in performance by our students. For example, we rejected some assessments with time scores below three minutes, which suggests at least some students did not put forth their best effort. Alternatively, the published ecology content instruments we used (Smith et al., 2019; Summers et al., 2018) may not have captured all dimensions of learning we are interested in capturing, such as critical thinking skills. In addition, students may be compensating temporarily for increased challenges and negative effects of the pandemic may be cumulative and become more apparent as the crisis drags on (Bono et al., 2020). At least one study found NLGs actually slightly increased the first semester of the pandemic (spring of 2020), despite going to an online format in a microbiology program that generally relies on in-person labs (Seitz & Rediske, 2021). Online courses can be more or less effective than in-person instruction depending on the course development and whether instructors can increase individual student-to-instructor contact time (e.g., Burger, 2015; Cavinato et al., 2021; Offir et al., 2008; Seitz & Rediske, 2021); this possibility of providing quality education in an online format should encourage educators. In this study, pre-pandemic, our courses already included strategies that would lessen pandemic strain, such as the availability of recorded mini-lectures and formative assessment online, as well as a synchronous portion of class twice weekly that consisted of active learning activities. This may have helped us retain learning gains in the face of pandemic challenges. Even if NLGs are truly not affected by the obstacles we surveyed, the obstacles might still affect motivation and persistence in the degree program (Yazdani et. al., 2021). We were unable to assess this in our current study. Thus, care should be taken when interpreting our results surrounding NLGs.
Potential Solutions
Students self-identified flexible assignment deadlines, quiet study spaces with good wifi, and more asynchronous material as potential solutions to these challenges. Flexibility has been highlighted as a solution in several studies; for example, resilience pedagogy is a strategy where resilience is incorporated into the design of the course, a focus of the teacher’s interactions, and resilience is modeled for students (Thurston et al. 2021 and references therein). We embrace the RAFT approach (resilient and flexible teaching) in which we incorporate flexibility in due dates, instructional methods, and assessment methods (Fabrey & Keith, 2021). We also strive to incorporate Universal Design for Instruction to make materials and assessments accessible to all students; for example, we work to improve cultural awareness and access for material for students who process information differently (e.g., visual or auditory impairment, etc.; Fabrey & Keith, 2021; Schwartzman, 2020). Our surveys indicated the importance of this flexibility as an approach to relieve pandemic-related issues; however, such strategies go beyond the pandemic to create a more inclusive learning environment for the broader demographic of student learners at all times.
Access to fast wifi and quiet study zones was the second most preferred solution. Nearly 15% of students “Often” struggled with the internet that had data limitations and 5.5% of students accessed the internet with a hotspot. The issue of nonexistent, spotty, expensive, and data-limited Internet and cell service is not unique to rural areas and Tribal lands in Utah (e.g., Gonzales et al., 2020). The “Broadband Progress Report” by the Federal Communications Commission indicates that 39% of the total rural U.S. population could not access basic fixed terrestrial broadband service and of those with service, almost 31% lacked access to speeds of 1 megabit/second for download (FCC 2016). This obstacle plagues many rural areas and is creating consistent barriers to education (Marietta & Marietta, 2021). Science, technology, engineering, and math (STEM) teaching resources that can be downloaded and completed offline with small document sizes can aid equal access to materials (Rhodes, 2021). Given these barriers, the use of phones as a primary means of engaging with course material is particularly worrisome. Some campuses at our institution have computers students can check out, but this is clearly not fulfilling the needs of all our students and some students may not even realize how using phones to access course material may negatively impact their learning. Additional research in surmounting this problem would be welcome.
Third, students requested more asynchronous material. This would aid flexibility in when students can access material. Students also requested more time with instructors and peer assistance groups during synchronous class time and ranked these options above more office hours or facilitating the formation of peer study groups outside of class. Schwartzman (2020) noted that required synchronous attendance can disproportionately negatively impact marginalized groups that may have less flexible work and caregiving schedules. However, good online pedagogy practices include more synchronous contact time, which leads to better student outcomes (e.g., Francescucci & Foster, 2013). Hence, we do not recommend lessening synchronous contact time. Instead, mitigation of the negative effects of synchronous meetings can be achieved by recording synchronous sessions and providing “make-up” opportunities for any points missed. Additionally, care should be taken to make synchronous sessions intrinsically valuable to students (that is, students want to attend rather than are coerced to attend; Cameron & Pierce, 1994; Deci et al., 1999; Wiersma, 1992). The value of synchronous course time can be aided by fostering a sense of community (video announcements, engaging discussions; e.g., Cavinato et al., 2021; Howard, 2015; Woods & Bliss; 2016) and including opportunities for formative assessment during the synchronous meetings (e.g., Dirks et al., 2014; Hake 1998ab; Handelsman et al., 2004; 2007).
In addition to course-level adjustments such as flexible assignment dates and more asynchronous material, university-wide solutions are needed. The PCA identified food and housing security as one of the greatest challenges. Of the solutions, 20% of students ranked food banks on campus as a high-priority, with an additional 35% ranking food banks as medium-priority. Given that half our students were employed yet still reported high scores on food and housing insecurity suggests our students may be underemployed or poorly paid. This suggests a requirement to work longer hours or more than preferred while attending school, which can have negative effects on learning (Geel & Backes-Gellner, 2012). Though the average score for this question may be lower than other solutions offered, it indicates a great need for added food security in over half of our students. Food assistance could allow underemployed or underpaid students to redirect more of their limited finances to housing. We are also quick to recognize that some solutions prioritized by a lower proportion of students may be important institutional priorities as they may assist, recruit, and retain a more diverse student body. For example, having lactation rooms and food banks on campus could lessen the burden on non-white females who face more obstacles than other groups. The same students who ranked food banks as a high-priority also ranked lactation rooms on campus as a high-priority. In addition, tailored advising and guidance about navigating college culture could aid first-generation students, who also face more challenges than other groups (Padron, 1992; Soria et al., 2020; Stebleton & Soria; 2013). Food banks, lactation rooms, and specialized advising may be available at the main campus and on some statewide campuses at our institution to varying degrees; however, many students may not be aware of these resources and expanded information campaigns may help.
Another challenge that institutions could address is caregiving; many of our students reported caregiving responsibilities increased during the pandemic. The childcare crisis in the United States existed before the pandemic and was then exacerbated by the pandemic (Petts et al., 2021, Boesch et. al., 2021). While reducing the spread of COVID-19 limited access to such services during the pandemic, we would be remiss not to note the critical importance caregiving services constitute for learners. Childcare consistently comes up in the literature as one of the biggest barriers for students (e.g., Gonchar, 1995; Moreau & Kerner 2015). Some statewide campuses at USU have access to childcare options for students including Brigham Early Care and Education Center, Jr. Aggies Academy on the Blanding Campus, and Little Aggies of Uintah Basin, but many campuses, regardless of size, do not. Age ranges, hours, expenses, and availability of these centers vary and it is unclear the degree to which they are meeting the childcare needs of USU students, on the main campus or statewide. One of us (SLB) personally chose a graduate school based on the availability of quality on-campus, sliding-scale childcare. Females (~ half our students) are often more affected by caregiving challenges and this trend has amplified during the pandemic (e.g., Petts et al., 2021; Sevilla & Smith, 2020; Zamarro & Prados, 2021); thus, institutions should find workarounds even in the face of the pandemic. Childcare is one supportive measure, but struggling parents of any sex can also be aided by mental health, food, and housing services (e.g., Manze et al., 2021) and simply educating students on what resources are already available (e.g., Sallee & Cox, 2019). Thus, there are several institutional-level resources universities can provide to help relieve stressors on students.
Recommendations for Future Studies
We first address logistical considerations for anyone embarking on similar research followed by conceptual suggestions for future studies. Of 66 students in our three classes combined, we were able to recover complete (all three surveys) and reliable (earnest attempt on ecology pre-post assessments) data from 48 students. Our response rate was unusually high, in part due to our small class sizes, the enticement of extra credit, and potentially the limited extracurricular distractions during the pandemic. One obstacle that we determined was the lack of incentive for extra credit in specific students who were satisfied with their grade (either low or high). Lucas et al. (2020) incentivized USU students with entry into a lottery for a gift card, but garnered fairly low response rates (6% to 3%). Nguyen et al. (2020) determined that students preferred monetary compensation (65%) or extra credit in courses (60%) over other incentive options. Pre- and post-tests can be a required activity to unlock later course material in an online learning system such as Canvas; however, this may lead to fewer honest attempts (e.g., choosing answers at random to complete the activity). The fact that we explicitly shared the value and usefulness of the survey may have resulted in a higher than expected response rate. One should strive for more universal and less potentially biased compensation incentives.
Our unique identifiers, or self-generated identification codes (SGICs), were difficult for students to remember. We noticed that several students could remember their birth month but were more challenged by the birth order (step-children, adopted children, recent birth of siblings, etc.) and their high school mascot (attending multiple schools, homeschooled, etc.). Another potential problem was with similar codes from several students who were from the same hometown with only one high school. The literature provides more stagnant response questions “(1) first two letters of your mother’s maiden name; (2) two-letter abbreviation for the state in which you were born; (3) first two letters of the name of the school where you began ninth grade; (4) your date of birth; (5) number of older siblings, alive or deceased; (6) natural hair color; (7) typical shoe size; (8) number of your mother’s siblings, alive or deceased; (9) first two letters of your father’s first name” (Little et al., 2021). Instead of creating a code, respondents answered a series of questions with some flexibility in the exact answers in matching (Little et al., 2021). At least five response questions need to be included and questions need to be (1) salient, (2) consistent, (3) non-sensitive, (4) easy to consistently format over repeated assessments, and (5) not easy to decode (Audette et al., 2020). A resource page with training in these nuances of ethnographic techniques, for example from USU’s Office of Empowering Teaching Excellence, could be very useful for faculty interested in expanding their research into the scholarship of teaching and learning.
In hindsight, a few additional questions would have benefited our obstacle survey. Instead of asking about evictions (especially given a moratorium on evictions), insight into the students’ financial fragility would have been gained by asking a Likert-scale question pertaining to financial security such as “How confident are you that you could come up with $2,000 if an unexpected need arose within the next month?” (Lusardi et al., 2011). Additional questions could have included asking about a sense of community support, family support, and support on campus for the students or general wellbeing (e.g., Warren & Bordoloi, 2020). Central to successful education in rural areas is the notion of “communality” as a source of strength, which “absorb[s] the pressures of life,” especially during challenging times (Marietta & Marietta, 2021). We note that several students who faced multiple obstacles appeared to “rise to the occasion” with high normalized learning gains (Figure 2A) and who may possibly attribute their resiliency to community, familial support, or other support mechanisms. However, our survey could not quantitate precisely how those students escaped the burden of the obstacles they experienced.
Our data represent a rare quantitative evaluation of how students self-report the change in obstacles to learning because of the pandemic. This, of course, is a snapshot of the perceptions of students in the first 5–9 months of the ongoing pandemic and educators should continue to monitor the situation. In the spirit of the scholarship of teaching and learning (SoTL; Shulman, 2001), educators should continue to quantitatively assess student learning in response to pedagogy changes and environmental factors such as the pandemic and also recognize that student needs are a shifting target. Further work on the response of students to the pandemic in terms of course NLGs, long term learning retention, program retention and persistence, recruitment or enrollment, and consequent career success is certainly needed.
Conclusion
The COVID-19 pandemic worsened challenges for students. We recommend continued tracking of challenges because the effects of the pandemic may be cumulative (Lee et al. 2021). Even pre-pandemic, our students faced significant obstacles to learning, thus evidence-based teaching strategies should be incorporated regardless of the state of the current pandemic. Based on what we learned here, we will continue to have flexible assignment dates, provide asynchronous materials with formative assessment, and provide synchronous meetings focused on active learning with the caveat to be mindful not to further exclude already marginalized groups, who may be more likely to miss some meetings. We have also implemented the following: create additional asynchronous material (particularly review materials and practice questions with keys), create peer assistance groups during class time to increase engagement and learning (e.g., Preszler, 2009; Smith et al., 2011; Szpunar et al., 2013; Wilson & Arendale, 2011), and explicitly encourage students to use a safe, quiet space to view course material with newer computers and fast wifi where they are free from distraction. Institutions can increase the ability of students to avail themselves of campus resources and increase their focus in academics by offering private study areas, additional advising for first-generation college students, childcare, food banks, and lactation rooms. These resources will help all students whether they are currently facing a global crisis, a personal crisis, or just everyday challenges. Evaluating the empirical data collected here demonstrates the ubiquity of obstacles. Some obstacles were severe –– several students reported feeling unsafe at home or running out of food to eat with no money to buy more. This reminds us as instructors to be patient always and accommodating whenever reasonable with students. Education is more than just exposure to course content; using kindness and community to foster a favorable learning environment is also important (Marietta & Marietta, 2021).
Acknowledgments
We would like to acknowledge that all USU courses are taught on the traditional lands of the Diné, Goshute, Paiute, Shoshone, and Ute Peoples past and present, and honor the land itself and the people who have stewarded it throughout the generations. We could not have accomplished this study without our student participants. We appreciate the advice of three anonymous reviewers that greatly improved our paper. We thank Summers and others for the development of the EcoEvo-MAPS survey for providing a baseline for our assessment of learning gains. Our deepest gratitude goes to the Green River where we float, reflect, and are inspired to educate ourselves and others in the field of ecology. We appreciate our families who tolerate our love of teaching and our long work hours.
References
Abdi, H., & Williams, L. J. (2010). Principal component analysis. Wiley Interdisciplinary Reviews: Computational Statistics, 2(4), 433-459.
Al-Mawee, W., Kwayu, K. M., & Gharaibeh, T. (2021). Student’s perspective on distance learning during COVID-19 pandemic: A case study of Western Michigan University, United States. International Journal of Educational Research Open, 2, 100080.
Ariely, D., & Wertenbroch, K. (2002). Procrastination, deadlines, and performance: Self-control by precommitment. Psychological Science, 13(3), 219-224.
Audette, L. M., Hammond, M. S., & Rochester, N. K. (2020). Methodological issues with coding participants in anonymous psychological longitudinal studies. Educational and Psychological Measurement, 80(1), 163-185.
Boesch, T., Grunewald, R., Nunn, R., & Palmer, V. (2021). Pandemic pushes mothers of young children out of the labor force. Federa, Reserve Bank of Minneapolis (Federal Reserve Bank of Minneapolis), 1-5.
Bono, G., Reil, K., & Hescox, J. (2020). Stress and wellbeing in urban college students in the US during the COVID-19 pandemic: Can grit and gratitude help? International Journal of Wellbeing, 10(3).
Breslow, N. E. (1996). Generalized linear models: checking assumptions and strengthening conclusions. Statistica Applicata, 8(1), 23-41.
Brewer, R., & Movahedazarhouligh, S. (2018). Successful stories and conflicts: A literature review on the effectiveness of flipped learning in higher education. Journal of Computer Assisted Learning, 34(4), 409-416.
Broadbent, J., & Poon, W. L. (2015). Self-regulated learning strategies & academic achievement in online higher education learning environments: A systematic review. The Internet and Higher Education, 27, 1-13.
Brodeur, A., Gray, D., Islam, A., & Bhuiyan, S. (2021). A literature review of the economics of COVID‐19. Journal of Economic Surveys, 35(4), 1007-1044.
Burger, B. (2015). Course delivery methods for effective distance science education: A case study of taking an introductory geology class online. In Interdisciplinary Approaches to Distance Teaching (pp. 104-117). Routledge.
Cameron, J., & Pierce, W. D. (1994). Reinforcement, reward, and intrinsic motivation: A meta-analysis. Review of Educational Research, 64, 363-423.
Cavinato, A. G., Hunter, R. A., Ott, L. S., & Robinson, J. K. (2021). Promoting student interaction, engagement, and success in an online environment. Analytical and Bioanalytical Chemistry, 413, 1513-1520.
Currie, J., & Thomas, D. (2001). Early test scores, school quality and SES: Longrun effects on wage and employment outcomes. In Worker Wellbeing in a Changing Labor Market. Emerald Group Publishing Limited.
Deci, E. L., Koestner, R. & Ryan, R. M. (1999). A meta-analytic review of experiments examining the effects of extrinsic rewards on intrinsic motivation. Psychological Bulletin, 125, 627.
Dirks, C., Wenderoth, M. P., & Withers, M. (2014). Assessment in the College Classroom. New York, New York: Freeman and Company.
Donnelly, R., & Patrinos, H. A. (2021). Learning loss during COVID-19: An early systematic review. Prospects, 1-9.
Fabrey, C., & Keith, H. (2021). Resilient and flexible teaching (RAFT): Integrating a whole-person experience into online teaching. In T. N., Thurston, K., Lundstrom, C., González (Eds.). Resilient Pedagogy: Practical Teaching Strategies to Overcome Distance, Disruption, and Distraction (pp. 114-129). Logan, UT: Empower Teaching Open Access Book Series.
FCC, Federal Communications Commission. Broadband Progress Report (2016). Retrieved from https://www.fcc.gov/reports-research/reports/broadband-progress-reports/2016-broadband-progress-report.
Francescucci, A., & Foster, M. (2013). The VIRI (virtual, interactive, real-time, instructor-led) classroom: The impact of blended synchronous online courses on student performance, engagement, and satisfaction. Canadian Journal of Higher Education, 43(3), 78-91.
Freeman, S., Eddy, S. L., McDonough, M., Smith, M. K., Okoroafor, N., Jordt, H., & Wenderoth, M. P. (2014). Active learning increases student performance in science, engineering, and mathematics. Proceedings of the National Academy of Sciences, 111, 8410-8415.
Geel, R., & Backes-Gellner, U. (2012). Earning while learning: When and how student employment is beneficial. Labour, 26(3), 313-340.
Gilbert, C., Siepser, C., Fink, A. E., & Johnson, N. L. (2021). Why LGBTQ+ campus resource centers are essential. Psychology of Sexual Orientation and Gender Diversity, 8(2), 245.
Gonchar, N. (1995). College-student mothers and on-site child care: Luxury or necessity? Children & Schools, 17(4), 226-234.
Gonzales, A. L., McCrory Calarco, J., & Lynch, T. (2020). Technology problems and student achievement gaps: A validation and extension of the technology maintenance construct. Communication Research, 47(5), 750-770.
Guàrdia, L., Maina, M., & Sangrà, A. (2013). MOOC design principles: A pedagogical approach from the learner’s perspective. eLearning Papers, 33, 1-6.
Hake, R. R. (1998a). Interactive-engagement versus traditional methods: a six-thousand-student survey of mechanics test data for introductory physics courses. American Journal of Physics, 66, 64-74.
Hake, R. R. (1998b). Interactive engagement methods in introductory physics mechanics courses. American Journal of Physics, 66, 1.
Handelsman, J., Ebert-May, D., Beichner, R., Bruns, P., Chang, A., DeHaan, R., Gentile, J., Lauffer, S., Stewart, J., Tilghman, S.M. & Wood, W.B. (2004). Scientific teaching. Science 304(5670), 521-522.
Handelsman, J., Miller, S. & Pfund, C. (2007). Scientific Teaching. New York, New York: W. H. Freeman and Company.
Howard, J. R. (2015). Discussion in the college classroom: Getting your students engaged and participating in person and online. San Francisco, CA: John Wiley & Sons, Inc.
Jacobs, J. A., & King, R. B. (2002). Age and college completion: A life-history analysis of women aged 15-44. Sociology of Education, 75(July), 211-230.
Jakubowski, T. D., & Sitko-Dominik, M. M. (2021). Teachers’ mental health during the first two waves of the COVID-19 pandemic in Poland. PloS One, 16(9), e0257252.
Johnson, G. M. (2006). Synchronous and asynchronous text-based CMC in educational contexts: A review of recent research. TechTrends, 50(4), 46-53.
Jolliffe, I. (2005). Principal component analysis. Encyclopedia of Statistics in Behavioral Science. San Francisco, CA: John Wiley & Sons, Inc.
Kimble-Hill, A. C., Rivera-Figueroa, A., Chan, B. C., Lawal, W. A., Gonzalez, S., Adams, M. R., Heard, G. L., Gazely, J. L., & Fiore-Walker, B. (2020). Insights gained into marginalized students access challenges during the COVID-19 academic response. Journal of Chemical Education, 97(9), 3391-3395.
Kramarczuk, K., Plane, J., & Atchison, K. (2021). First-generation undergraduate women and intersectional obstacles to pursuing post-baccalaureate computing degrees. In 2021 Conference on Research in Equitable and Sustained Participation in Engineering, Computing, and Technology (RESPECT) IEEE. pp. 1-8.
Ladson-Billings, G. (2021). I’m here for the hard re-set: Post pandemic pedagogy to preserve our culture. Equity & Excellence in Education, 54(1), 68-78.
Lang, J. M. (2020). Distracted: Why Students Can’t Focus and What You Can Do about it. New York, NY: Basic Books, Hachette Book Group.
Larsen, D. P., Butler, A. C., & Roediger III, H. L. (2009). Repeated testing improves long-term retention relative to repeated study: a randomized controlled trial. Medical Education, 43, 1174-1181.
Lee, J., Solomon, M., Stead, T., Kwon, B., & Ganti, L. (2021). Impact of COVID-19 on the mental health of US college students. BMC Psychology, 9(1), 1-10.
Little, M. A., Bonilla, G., McMurry, T., Pebley, K., Klesges, R. C., & Talcott, G. W. (2021). The feasibility of using self-generated identification codes in longitudinal research with military personnel. Evaluation & the Health Professions, DOI 01632787211031625, 1-8.
Lucas, L. K., Hunter, F. K., & Gompert, Z. (2020). Effect of Three Classroom Research Experiences on Science Attitudes. Journal on Empowering Teaching Excellence, 4(2), 8.
Lusardi, A., Schneider, D. J., & Tufano, P. (2011). Financially fragile households: Evidence and implications (No. 17072). Cambridge, MA: National Bureau of Economic Research Working Paper Series.
Manze, M., Watnick, D., & Freudenberg, N. (2021). How do childcare and pregnancy affect the academic success of college students? Journal of American College Health, 1-8.
Marietta, G., & Marietta, S. (2021). Rural Education in America: What Works for Our Students, Teachers, and Communities. Cambridge, MA: Harvard Education Press.
McKnight, P. E., & Najab, J. (2010). Mann‐Whitney U Test. The Corsini Encyclopedia of Psychology, 1-1.
Michinov, N., Brunot, S., Le Bohec, O., Juhel, J., & Delaval, M. (2011). Procrastination, participation, and performance in online learning environments. Computers & Education, 56(1), 243-252.
Moore, S. E., Wierenga, K. L., Prince, D. M., Gillani, B., & Mintz, L. J. (2021). Disproportionate impact of the COVID-19 pandemic on perceived social support, mental health and somatic symptoms in sexual and gender minority populations. Journal of Homosexuality, 68(4), 577-591.
Moreau, M. P., & Kerner, C. (2015). Care in academia: An exploration of student parents’ experiences. British Journal of Sociology of Education, 36(2), 215-233.
Morgan, H. (2020). Best practices for implementing remote learning during a pandemic. The Clearing House: A Journal of Educational Strategies, Issues and Ideas, 93(3), 135-141.
Mustafa, N. (2020). Impact of the 2019–20 coronavirus pandemic on education. International Journal of Health Preferences Research, 1-12.
Nguyen, V., Kaneshiro, K., Nallamala, H., Kirby, C., Cho, T., Messer, K., Zahl, S., Hum, J., Modrzakowski, M., Atchley, D., Ziegler, D., Pipitone, O., Lowery, J. W., & Kisby, G. (2020). Assessment of the research interests and perceptions of first-year medical students at 4 colleges of osteopathic medicine. Journal of Osteopathic Medicine, 120(4), 236-244.
Núñez, A. M. (2014). Employing multilevel intersectionality in educational research: Latino identities, contexts, and college access. Educational Researcher, 43(2), 85-92.
Offir, B., Lev, Y., & Bezalel, R. (2008). Surface and deep learning processes in distance education: Synchronous versus asynchronous systems. Computers & Education, 51(3), 1172-1183.
Ozan, O., & Ozarslan, Y. (2016). Video lecture watching behaviors of learners in online courses. Educational Media International, 53(1), 27-41.
Padron, E. J. (1992). The challenge of first-generation college students: A Miami-Dade perspective. New Directions for Community Colleges, 80, 71-80.
Petts, R. J., Carlson, D. L., & Pepin, J. R. (2021). A gendered pandemic: Childcare, homeschooling, and parents’ employment during COVID-19. Gender, Work & Organization, 28, 515-534.
Preszler, R. W. (2009). Replacing lecture with peer-led workshops improves student learning. CBE-Life Sciences Education, 8, 182-192.
Preszler, R. W., Dawe, A., Shuster, C. B., & Shuster, M. (2007). Assessment of the effects of student response systems on student learning and attitudes over a broad range of biology courses. CBE-Life Sciences Education, 6, 29-41.
Ro, H. K., & Loya, K. I. (2015). The effect of gender and race intersectionality on student learning outcomes in engineering. The Review of Higher Education, 38(3), 359-396.
Roediger, H. L. III, & Karpicke, J. D. (2006). The power of testing memory: basic research and implications for educational practice. Perspectives on Psychological Science, 1, 181-210.
Roediger, H. L. III, Putnam, A. L., & Smith, M. A. (2011). Ten benefits of testing and their applications to educational practice. Psychology of Learning and Motivation-Advances in Research and Theory, 55, 1.
Reyes, M. V. (2020). The disproportional impact of COVID-19 on African Americans. Health and Human Rights, 22(2), 299.
Rhodes, A. (2021). Lowering barriers to active learning: a novel approach for online instructional environments. Advances in Physiology Education, 45(3), 547-553.
Sallee, M. W., & Cox, R. D. (2019). Thinking beyond childcare: Supporting community college student-parents. American Journal of Education, 125(4), 621-645.
Schwartzman, R. (2020). Performing pandemic pedagogy. Communication Education, 69(4), 502-517.
Seitz, H., & Rediske, A. (2021). Impact of COVID-19 Curricular shifts on learning gains on the Microbiology for Health Sciences Concept Inventory. Journal of Microbiology & Biology Education, 22(1), ev22i1-2425.
Sevilla, A., & Smith, S. (2020). Baby steps: the gender division of childcare during the COVID-19 pandemic. Oxford Review of Economic Policy, 36(Supplement_1), S169-S186.
Shulman, L. (2001). From Minsk to Pinsk: Why a scholarship of teaching and learning? Journal of the Scholarship of Teaching and Learning, 1(1), 48-53.
Smith, M. K., Walsh, C., Holmes, N. G., & Summers, M. M. (2019). Using the Ecology and Evolution-Measuring Achievement and Progression in Science assessment to measure student thinking across the Four-Dimensional Ecology Education framework. Ecosphere, 10(9), e02873.
Smith, M. K., Wood, W. B., Krauter, K., & Knight, J. K. (2011). Combining peer discussion with instructor explanation increases student learning from in-class concept questions. CBE-Life Sciences Education, 10, 55-63.
Sokal, L., Trudel, L. E., & Babb, J. (2020). Canadian teachers’ attitudes toward change, efficacy, and burnout during the COVID-19 pandemic. International Journal of Educational Research Open, 1, 100016.
Soria, K. M., Horgos, B., Chirikov, I., & Jones-White, D. (2020). First-generation students’ experiences during the COVID-19 pandemic. Berkeley, CA: Student Experience in the Research University (SERU) Consortium.
Stebleton, M., & Soria, K. (2013). Breaking down barriers: Academic obstacles of first-generation students at research universities. Learning Assistance Review, 17(2), 7-19.
Strayhorn, T. L., & DeVita, J. M. (2010). African American males’ student engagement: A comparison of good practices by institutional type. Journal of African American Studies, 14, 87-105.
Summers, M. M., Couch, B. A., Knight, J. K., Brownell, S. E., Crowe, A. J., Semsar, K., Wright, C. D., & Smith, M. K. (2018). EcoEvo-MAPS: an ecology and evolution assessment for introductory through advanced undergraduates. CBE—Life Sciences Education, 17(2), ar18.
Szpunar, K. K., Khan, N. Y., & Schacter, D. L. (2013). Interpolated memory tests reduce mind wandering and improve learning of online lectures. Proceedings of the National Academy of Sciences, 110, 6313-6317.
Tanner, K. D. (2009). Talking to learn: why biology students should be talking in classrooms and how to make it happen. CBE-Life Sciences Education, 8, 89-94.
Thurston, T. N., Lundstrom, K., González, C. (Eds.). (2021). Resilient Pedagogy: Practical Teaching Strategies to Overcome Distance, Disruption, and Distraction. Logan, UT: Empower Teaching Open Access Book Series.
US Department of Agriculture. (2012). US Adult Food Security Survey Module: Three-Stage Design, with Screeners. Retrieved from https://www.ers.usda.gov/topics/food-nutrition-assistance/food-security-in-the-us/survey-tools/#household.
Wang, X., Hegde, S., Son, C., Keller, B., Smith, A., & Sasangohar, F. (2020). Investigating mental health of US college students during the COVID-19 pandemic: cross-sectional survey study. Journal of Medical Internet Research, 22(9), e22817.
Warren, M. A., & Bordoloi, S. (2020). When COVID-19 exacerbates inequities: The path forward for generating wellbeing. International Journal of Wellbeing, 10(3).
Weber, E. (2009). Quantifying student learning: how to analyze assessment data. Bulletin of the Ecological Society of America, 90(4), 501-511.
Wiersma, U. J. (1992). The effects of extrinsic rewards in intrinsic motivation: A meta‐analysis. Journal of Occupational and Organizational Psychology, 65, 101-114.
Wilson, W. L., & Arendale, D. R. (2011). Peer educators in learning assistance programs: Best practices for new programs. New Directions for Student Services, 133, 41-53.
Wood, W. B. (2009). Innovations in teaching undergraduate biology and why we need them. Annual Review Cell and Developmental Biology, 25, 93-112.
Woods, K., & Bliss, K. (2016). Facilitating Successful Online Discussions. Journal of Effective Teaching, 16(2), 76-92.
Yamagata-Lynch, L. C. (2014). Blending online asynchronous and synchronous learning. International Review of Research in Open and Distributed Learning, 15(2), 189-212.
Yaghi, A. (2022). Impact of online education on anxiety and stress among undergraduate public affairs students: A longitudinal study during the COVID-19 pandemic. Journal of Public Affairs Education, 28(1), 91-108.
Yazdani, N., McCallen, L. S., Hoyt, L. T., & Brown, J. L. (2021). Predictors of economically disadvantaged vertical transfer students’ academic performance and retention: A scoping review. Journal of College Student Retention: Research, Theory & Practice. DOI 15210251211031184.
Zamarro, G., & Prados, M. J. (2021). Gender differences in couples’ division of childcare, work and mental health during COVID-19. Review of Economics of the Household, 19(1), 11-40.