Part I: Sampling, Data Collection, & Analysis in Quantitative Research
In this module, we will focus on how quantitative research collects and analyzes data, as well as methods for obtaining sample population.
- Levels of Measurement
- Reliability and Validity
- Population and Samples
- Common Data Collection Methods
- Data Analysis
- Statistical Significance versus Clinical Significance
Objectives:
Part I:
- Describe levels of measurement
- Describe reliability and validity as applied to critical appraisal of research
- Differentiate methods of obtaining samples for population generalizability
- Describe common data collection methods in quantitative research
Part II:
- Describe various data analysis methods in quantitative research
- Differentiate statistical significance versus clinical significance
Levels of measurement
Once researchers have collected their data (we will talk about data collection later in this module), they need methods to organize the data before they even start to think about statistical analyses. Statistical operations depend on a variable’s level of measurement. Think about this similarly to shuffling all of your bills in some type of organization before you pay them. With levels of measurement, we are precisely recording variables in a method to help organize them.
There are four levels of measurement:
Nominal: The data can only be categorized
Ordinal: The data can be categorized and ranked
Interval: The data can be categorized, ranked, and evenly spaced
Ratio: The data can be categorized, ranked, even spaced, and has a natural zero
Going from lowest to highest, the 4 levels of measurement are cumulative. This means that they each take on the properties of lower levels and add new properties.
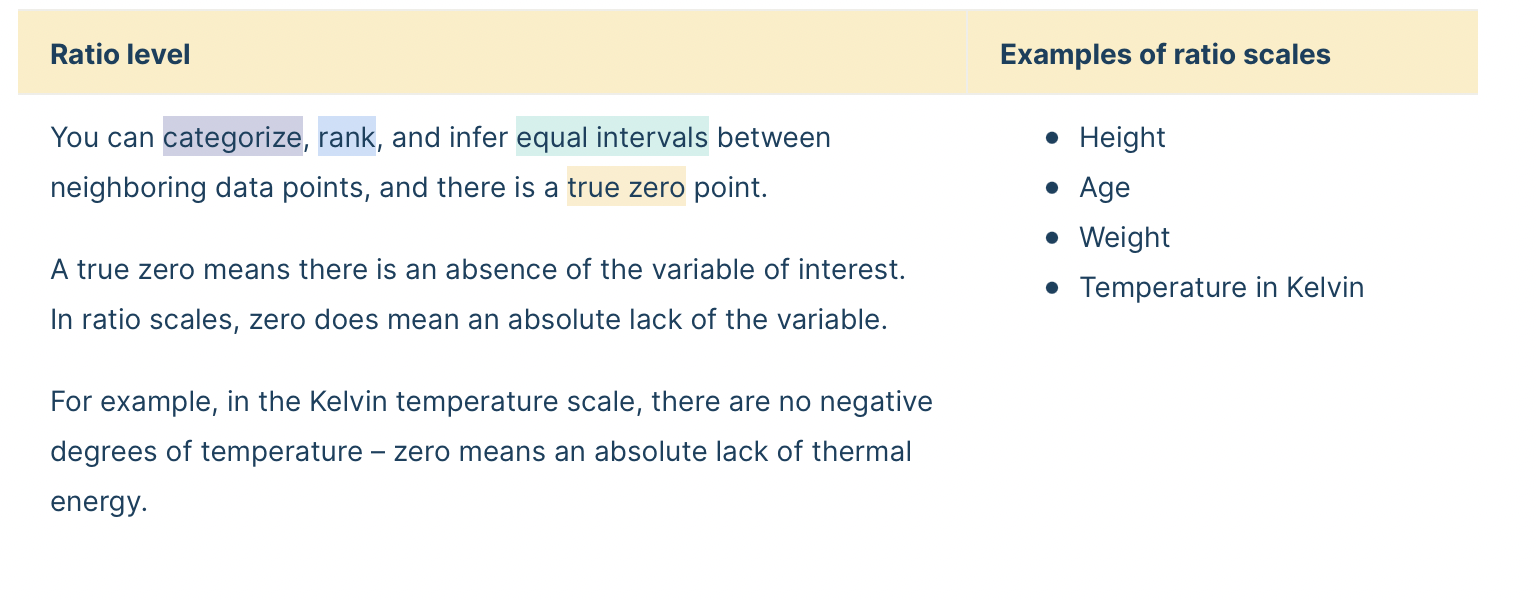
Why are levels of measurement important?
The level at which a researcher measures a variable determines how he/she can analyze the data.
The different levels limit which descriptive statistics can be used to get an overall summary of the data, and which type of inferential statistics can be performed on the data to support or refute the hypothesis.
In many cases, the variables can be measured at different levels, so the researcher has to choose the level of measurement they will use before data collection begins.
Hot Tip! How can you tell a variable’s measurement level?
- A variable is nominal if the values could be interchanged (e.g. 1 = male, 2 = female OR 1 = female, 2 = male).
- A variable is ordinal if there is a quantitative ordering of values AND if there are a small number of values (e.g. excellent, good, fair, poor).
- A variable is usually considered interval if it is measured with a composite scale or test.
- A variable is ratio level if it makes sense to say that one value is twice as much as another (e.g. 100 mg is twice as much as 50 mg) (Polit & Beck, 2021).
Reliability and Validity as Applied to Critical Appraisal of Research
Reliability measures the ability of a measure to consistently measure the same way. Validity measures what it is supposed to measure. Do we have the need for both in research? Yes! If a variable is measured inaccurately, the data is useless. Let’s talk about why.
For example, let’s set out to measure blood glucose for our study. The validity is how well the measure can determine the blood glucose. If we used a blood pressure cuff to measure blood glucose, this would not be a valid measure. If we used a blood glucose meter, it would be a more valid measure. It does not stop there, however. What about the meter itself? Has it been calibrated? Are the correct sticks for the meter available? Are they expired? Does the meter have fresh batteries? Are the patient’s hands clean?
Reliability wants to know: Is the blood glucose meter measuring the same way, every time?
Validity is asking, “Does the meter measure what it is supposed to measure?” Construct validity: Does the test measure the concept that it’s intended to measure? Content validity: Is the test fully representative of what it aims to measure? Face validity: Does the content of the test appear to be suitable to its aims?
Term |
Definition |
Importance |
Application |
Reliability |
Measures the ability of a measure to |
This is important for consistent measures of a construct.
|
For example, when measuring a patient’s blood pressure, the blood pressure cuff should consistently measure in the same way. So, when doing every 15-minute vital signs after surgery, the blood pressure cuff should measure consistently every 15 minutes.
|
Validity |
Measures the concept it is |
This is important to be able to measure the intended construct. |
For example, a measure of critical thinking is an accurate measure of critical thinking and not expert practice.
Another example: a measure of stress level should measure stress level, not pain level. |
Leibold, 2020
Obtaining Samples for Population Generalizability
In quantitative research, a population is the entire group that the researcher wants to draw conclusions about.
A sample is the specific group that the researcher will actually collect data from. A sample is always a much smaller group of people than the total size of the population. For example, if we wanted to investigate heart failure, there would be no possible way to measure every single human with heart failure. Therefore, researchers will attempt to select a sample of that large population which would most likely reflect (AKA: be a representative sample) the larger population of those with heart failure. Remember, in quantitative research, the results should be generalizable to the population studied.
There is a lot of confusion with students (and even some researchers!) when they refer to “random assignment” versus “random sampling”. Random assignment is a signature of a true experiment. This means that if participants are not truly randomly assigned to intervention groups, then it is not a true experiment. Remember, random sampling is a technique used to select individuals from a larger population to be included in a study. The key characteristic of random sampling is that every member of the population has an equal chance of being selected. Random assignment is a process used in experimental research to assign participants to different groups or conditions (e.g., treatment vs. control) in a way that each participant has an equal chance of being placed in any group.
A researcher will specify population characteristics through eligibility criteria. This means that they consider which characteristics to include (inclusion criteria) and which characteristics to exclude (exclusion criteria).
Inclusion and exclusion criteria are essential for defining who is eligible to participate in a study. Establishing inclusion and exclusion criteria for study participants is a standard, required practice when designing high-quality research protocols. Inclusion criteria are defined as the key features of the target population that the investigators will use to answer their research question (Boswell & Cannon, 2022). Typical inclusion criteria include demographic, clinical, and geographic characteristics.
For example, what might be your criteria for including someone from participation? Are any criteria based on age, gender, race, ethnicity, sexual orientation, origin, type and stage of disease, the subject’s previous treatment history, and the presence or absence (as in the case of the “healthy” or “control” subject) of other medical, psychosocial, or emotional conditions? If so, justify. For example, if you are doing a study on pregnant persons, will you exclude men (most likely, you will)? If you exclude men, even if it seems obvious, this needs to be determined and stated.
- Inclusion Criteria: These are the characteristics that participants must have to be included in the study. Inclusion criteria help ensure that the sample is relevant to the research question and that the findings will be applicable to the population of interest. For example, in a study on diabetic patients, inclusion criteria might include being diagnosed with Type 2 diabetes and being over the age of 18.
- Exclusion Criteria: These are the characteristics that disqualify potential participants from the study. Exclusion criteria are used to remove individuals who might confound the results or who do not fit the study’s focus. Continuing the example above, exclusion criteria might include pregnant patients or other chronic conditions that could interfere with the study outcomes.
For example, if we were studying chemotherapy in breast cancer subjects, we might specify:
- Inclusion Criteria: Postmenopausal women between the ages of 45 and 75 who have been diagnosed with Stage II breast cancer.
- Exclusion Criteria: Abnormal renal function tests since we are studying a combination of drugs that may be nephrotoxic. Renal function tests are to be performed to evaluate renal function and the threshold values that would disqualify the prospective subject is serum creatinine above 1.9 mg/dl.
Sampling Designs:
There are two broad classes of sampling in quantitative research: Probability and nonprobability sampling.
Probability sampling: As the name implies, probability sampling means that each eligible individual has a random chance (same probability) of being selected to participate in the study.
There are three types of probability sampling:
Simple random sampling: Every eligible participant is randomly selected (e.g. drawing from a hat).
Stratified random sampling: Eligible population is first divided into two or more strata (categories) from which randomization occurs (e.g. pollution levels selected from restaurants, bars with ordinances of state laws, and bars with no ordinances).
Systematic sampling: Involves the selection of every __th eligible participant from a list (e.g. every 9th person).
Nonprobability sampling: In nonprobability sampling, eligible participants are selected using a subjective (non-random) method.
There are four types of nonprobability sampling:
Convenience sampling: Participants are selected for inclusion in the sample because they are the easiest for the researcher to access. This can be due to geographical proximity, availability at a given time, or willingness to participate in the research.
Quota sampling: Participants are from a very tailored sample that’s in proportion to some characteristic or trait of a population. For example, the researcher could divide a population by the state they live in, income or education level, or sex. The population is divided into groups (also called strata) and samples are taken from each group to meet a quota.
Purposive sampling: A group of non-probability sampling techniques in which units are selected because they have characteristics that the researcher needs in their sample. In other words, units are selected “on purpose” in purposive sampling.
Snowball sampling: Existing study participants recruit future participants from their acquaintances or networks. It is often used in studies where the population is hard to reach.
And, a 5th type: Consecutive sampling: A sampling technique in which every subject meeting the criteria of inclusion is selected until the required sample size is achieved. Consecutive sampling is defined as a nonprobability technique where samples are picked at the ease of a researcher more like convenience sampling, only with a slight variation. Here, the researcher selects a sample or group of people, conducts research over a period, collects results, and then moves on to another sample.
The goal in sampling is that it is a representative sample that is similar to the larger population. A representative sample should resemble the population in key demographic, behavioral, or other relevant characteristics. This ensures that the findings from the sample can be extrapolated to the population. The sample must be large enough to capture the diversity of the population and minimize sampling error (the difference between the sample results and the actual population parameters). A small sample might not adequately represent the population, especially in terms of its variability. To create a representative sample, the selection process must be unbiased, meaning that no specific group is more or less likely to be included than others. Bias in the selection process can lead to an unrepresentative sample, which will distort the study results.
Common Data Collection Methods in Quantitative Research
There are various methods that researchers use to collect data for their studies. For nurse researchers, existing records are an important data source. Researchers need to decide if they will collect new data or use existing data. There is also a wealth of clinical data that can be used for non-research purposed to help answer clinical questions.
Let’s look at some general data collection methods and data sources in quantitative research.
Existing data could include medical records, school records, corporate diaries, letters, meeting minutes, and photographs. These are easy to obtain do not require participation from those being studied.
Collecting new data:
Let’s go over a few methods in which researcher can collect new data. These usually requires participation from those being studied.
Self-reports can be obtained via interviews or questionnaires. Closed-ended questions can be asked (“Within the past 6 months, were you ever a member of a fitness gym?” Yes/No) or open-ended questions such as “Why did you decide to join a fitness gym?” Important to remember (this sometimes throws students off) is that conducting interviews and questionnaires does not mean it is qualitative in nature! Do not let that throw you off in assessing whether a published article is quantitative or qualitative. The nature of the questions, however, may help to determine the type of research (quantitative or qualitative), as qualitative questions deal with ascertaining a very organic collection of people’s experiences in open-ended questions.
Advantages of questionnaires (compared to interviews):
- Questionnaires are less costly and are advantageous for geographically dispersed samples.
- Questionnaires offer the possibility of anonymity, which may be crucial in obtaining information about certain opinions or traits.
Advances of interviews (compared to questionnaires):
- Higher response rates
- Appropriate for more diverse audiences
- Some people cannot fill out a questionnaire.
- Opportunities to clarify questions or to determine comprehension
- Opportunity to collect supplementary data through observation
Psychosocial scales are often utilized within questionnaires or interviews. These can help to obtain attitudes, perceptions, and psychological traits.
Likert Scales:
- Consist of several declarative statements (items) expressing viewpoints
- Responses are on an agree/disagree continuum (usually five or seven response options).
- Responses to items are summed to compute a total scale score.
Visual Analog Scale:
- Used to measure subjective experiences (e.g., pain, nausea)
- Measurements are on a straight line measuring 100 mm.
- End points labeled as extreme limits of sensation
Observational Methods include the observation method of data collection involves seeing people in a certain setting or place at a specific time and day. Essentially, researchers study the behavior of the individuals or surroundings in which they are analyzing. This can be controlled, spontaneous, or participant-based research.
When a researcher utilizes a defined procedure for observing individuals or the environment, this is known as structured observation. When individuals are observed in their natural environment, this is known as naturalistic observation. In participant observation, the researcher immerses himself or herself in the environment and becomes a member of the group being observed.
Biophysiologic Measures are defined as ‘those physiological and physical variables that require specialized technical instruments and equipment for their measurement’. Biophysiological measures are the most common instruments for collecting data in medical science studies. To collect valid and reliable data, it is critical to apply these measures appropriately.
- In vivo refers to when research or work is done with or within an entire, living organism. Examples can include studies in animal models or human clinical trials.
- In vitro is used to describe work that’s performed outside of a living organism. This usually involves isolated tissues, organs, or cells.
Let’s watch a video about Sampling and Data Collection that I made a couple of years ago.
Critical Appraisal! Quantitative Sampling and Data Collection:
- Did the researchers use the best method of capturing study phenomena (i.e., self-reports, observation, biomarkers)?
- If self-report methods were used, did the researchers make good decisions about the specific methods used to solicit information (e.g., in-person interviews, Internet questionnaires, and so on)? Were composite scales used? If not, should they have been?
- If observational methods were used, did the report adequately describe what the observations entailed and how observations were sampled? Were risks of observational bias addressed? Were biomarkers used in the study, and was this appropriate?
- Did the report provide adequate information about data collection procedures (e.g., the training of the data collectors)?
- Did the report offer evidence of the reliability of measures? Did the evidence come from the research sample itself, or was it based on other studies? If reliability was reported, which estimation method was used? Was the reliability sufficiently high?
- Did the report offer evidence of the validity of the measures? If validity information was reported, which validity approach was used?
- If there was no reliability or validity information, what conclusion can you reach about the quality of the data in the study?
References & Attribution
“Magnifying glass” by rawpixel licensed CC0
“Orange flame” by rawpixel licensed CC0
Bhandari, P. (2022). Levels of Measurement | Nominal, Ordinal, Interval and Ratio. Scribbr. https://www.scribbr.com/statistics/levels-of-measurement/
Polit, D. & Beck, C. (2021). Lippincott CoursePoint Enhanced for Polit’s Essentials of Nursing Research (10th ed.). Wolters Kluwer Health.