Does Increased Online Interaction Between Instructors and Students Positively Affect a Student’s Perception of Quality for an Online Course?
Jennifer Hunter, Ph.D. and Brayden Ross
Abstract
Online education is increasing as a solution to manage increasing enrollment numbers at higher education institutions. Intentionally and thoughtfully constructed courses allow students to improve performance through practice and self-assessment and instructors benefit from improving consistency in providing content and assessing process, performance, and progress.
The purpose of this study was to examine the effect of student to instructor interaction on the student’s perception of quality for an online course. “Does increased online interaction between instructors and students positively affect a student’s perception of quality for an online course?”
The study included over 1200 courses over a three year time period in a public, degree-granting higher education institution. The top two findings of the case study included an overall linear relationship between interactions per student and overall perception of quality in addition to a statistically significant relationship between interactions per student and quality-of-course scoring by students using linear regression with fixed effects for colleges. These findings were significant at the 99% level.
The implications resulting from this study, based on the data, can be used by administrators and faculty to create high-quality online courses providing students a sense of belonging in an online learning environment.
Introduction (Statement of the Problem)
With online learning enrollments growth (Poll, Widen, & Weller, 2014) outpacing traditional higher education (Allen & Seaman, 2015), it is becoming important to focus on the design and delivery of online courses (CHLOE, 2017; Kearns, 2012; Meyer, 2014). However, undergraduate curriculum has remained essentially unchanged during the last half-century (Bass 2012). The move to online courses opens up possibilities, including but not limited to personalized education in the online realm (Weld, Adar, Chilton, Hoffmann, Horvitz, Koch, Landay, Lin, & Mausam, 2012).
A question often asked in the literature, “What can administrators do to increase an effective online environment” (Jaggars, Edgecombe, & Stacey, 2013) goes unanswered when related to pedagogy, although many research articles answer the question related to technology (Huneycutt, 2013; Hogg & Limicky, 2012; Grabe & Holfeld, 2014). Related questions include; (a) how an online class is effectively monitored while it is in session, (b) how many days a professor should participate in the asynchronous learning environment, (c) when feedback should be provided and what constitutes substantive feedback, (d) what are the appropriate level of interactions with students, (e) how course materials are aligned and scaffolded with accreditation standards (such as ISLLC and CCSSO), and finally (f) what constitutes meeting the university contract hour per week (B. Reynolds, personal communication, January 04, 2017). This study attempts to answer question (d) what are the appropriate level of interactions with students. The focus was on purposed, meaningful interactions (Kuh & O’Donnell, 2013), as one student from the institution stated: “too much student-teacher interaction puts me in a position where I feel like the attention is negative from the professor” (E. Buchanan, personal communication, January 5, 2018). A positive correlation between instructor presence in discussion forums and higher student grades was reported in one study (Cranney, Alexander, Wallace, Alfano, 2011).
Research Question/Context
“Does increased online interaction between instructors and students positively affect a student’s perception of quality for an online course?” The study included over 1200 courses over a three-year time period in a public, degree-granting, higher education institution. The top two findings of the case study included an overall linear relationship between interactions per student and overall perception of quality in addition to a statistically significant relationship between interactions per student and quality-of-course scoring by students using linear regression with fixed effects for colleges. These findings were significant at the 99% level.
Literature Review
The last decade has seen an emergence of social constructivism as a learning theory focused on knowledge distributed socially (Hunter, 2017). One element of social theory is community (Taylor & Hamdy, 2013), which included interaction. Social interaction positively affects the learning process (Baker, 2011). A social constructivist learning theory focusing on student interactions, whereas the social constructivist teaching theory concentrates on the interaction between the student and teacher with an emphasis on student engagement with the content (Bryant & Bates, 2015; Moreillon, 2015).
The importance of interactions in the online learning environment is the focus of many studies (Brinthaupt, Fisher, Gardner, & Raffo, 2011; Hogg & Lomicky, 2012; Watts, 2016). The quantity of interaction and the quality are both important elements to perceived interaction (Brinthaupt, et al., 2011). One study’s findings include the amount of time spent studying online was only beneficial if some form of interaction was part of the study process (Castano-Munoz, Duart, & Vinuesa, 2014). Interaction can be synchronous or asynchronous. Typical asynchronous interaction in online courses occurs with discussion boards (Kleinsasser & Hong, 2016).
Interaction can be instructor to learner, learner to learner, and learner to content (Baker, 2011; Goldman, 2011) with the first two types affecting social and community aspects of learning. The results of one study on student satisfaction in online courses found interaction with the instructor (instructor to learner) was a significant contributor (Goldman, 2011, Bonfiglio, O’Bryan, Palavecino, Willibey, 2016) to student learning.
Interactions with instructors can increase academic achievement and student satisfaction with college courses (Barkley et al., 2014). The lack of instructor to learner and learner to learner interaction in an online course has led educators and researchers to seek effective methods for keeping students engaged in an online learning environment (Findlay-Thompson & Mombourquette; 2014; Watts, 2016). The advantage of current education technology allows for engaged students (Ertmer & Newby, 2013), creating opportunities for instructors to facilitate student participation and interaction (Stear & Mensch, 2012).
Data collection/method
Data for this study was extracted from the institution’s online learning management system. The data was compiled together including assignment submission comments and conversation messages broken up by course, department and college.
For this study, interactions were counted at a course level. An “interaction” will hereafter be denoted as an instructor making a comment on a student’s submission or an instructor responding to a student’s message or sending a message to a student. Mass messages (i.e., sent to the entire class) were counted as one interaction, as opposed to, for example, 30 (1 per student). This was done to ensure the interactions occurring between students and teachers were personalized, rather than mass-produced.
For this institution, an end-of-course standardized survey is conducted to determine student experience, quality of instruction, and numerous other measures. The final scores are based on an average of all the section scores in the survey and can range anywhere from 1 (lowest) to just above 5 (highest). For online courses at this institution, these survey scores are only available if there were enough responses to provide a comprehensive survey sample of the course, in this case, a minimum of five students in the course with at least three students responding. Any and all courses not meeting this requirement are omitted from the data. This omission also accounts for outliers, which might otherwise affect the analysis. Courses with high interaction counts and less than five students are not included due to lack of substantial survey responses, thereby removing outliers from the dataset and ensuring accuracy of prediction.
In addition, as a robustness check to ensure trends were similar across time, we examined semester data over the last three complete years (2015-2018). The semesters include summer, fall, and spring.
Results
Examining the scatter plot in Figure 1 and its best fit line, we can infer the relationship between interactions per student and quality survey score is positive overall three years. This shows increases in meaningful interaction increase the perceived quality of a course by a student across the entire time-period examined. It should be noted that there is a clustering of courses with high evaluation survey scores and low interaction count. This is most likely due to the student’s perceived necessity of interaction with the teacher being minimal, or rather the fact that interaction takes place in differing communication methods outside of the learning management system. For these reasons, it is important to keep in mind that action plans should be implemented on a case by case basis predicated on previous data, which may or may not prove that student preference favors or warrants more interaction.
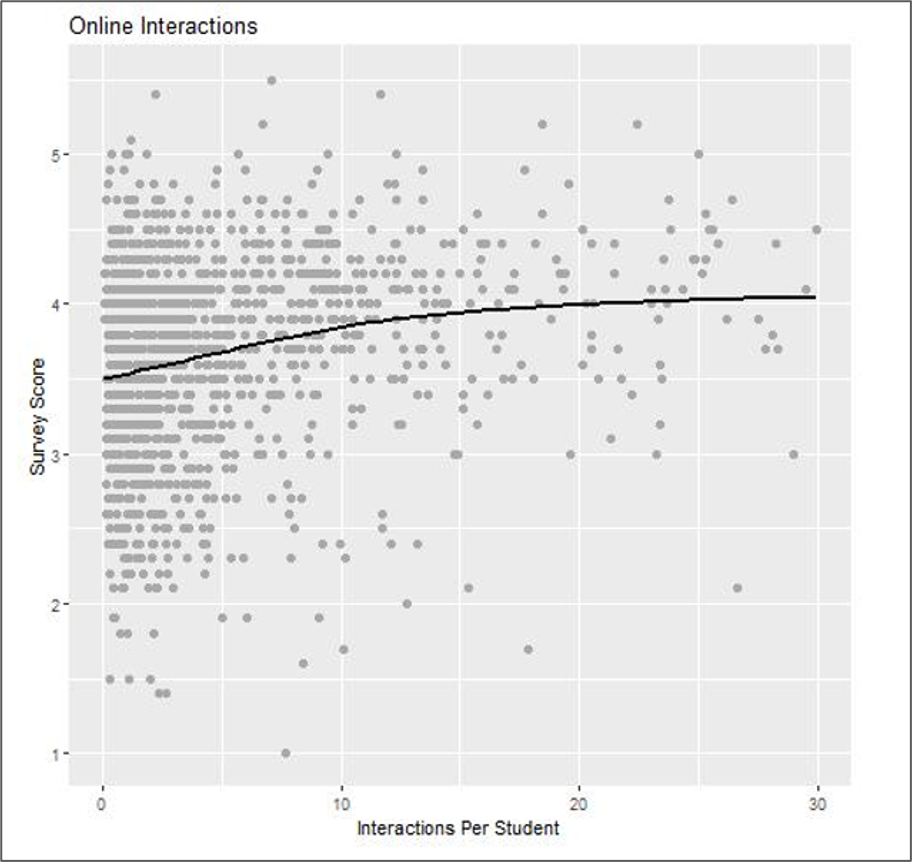
To determine if the relationship is indeed linear and statistically significant, we used a linear regression model with fixed effects for colleges (controlling for College of Business, College of Education, etc.), with the dependent variable being Survey Scores and the independent being interactions per student.
Table 1 (below) shows the results for the linear regression model using fixed effects for college. Interactions per student are statistically significant at the 99% level, showing that for each additional interaction per student, an instructor can increase their survey scores by .01 points. The R-squared value shows the model explains approximately 25% of the variation in Survey Score with the provided variables for this dataset.
Dependent variable: | |
---|---|
Interactions Per Student | Survey Score |
0.011*** | |
(0.003) | |
Observations | 1,264 |
R2 | 0.253 |
Adjusted R2 | 0.247 |
Residual Std. Error | 0.573 (df = 1253) |
Linear regression w/ Fixed Effects for Colleges |
Limitations
The main limitation in the model and the results is the overall perception of quality of the course. There is still approximately 75% of the variation in survey scores left unexplained. Other factors affect quality of a course, such as the depth of coursework, difficulty, ease of access to instructors, speed of response to student questions, and teacher-student compatibility. These factors likely fill that missing explanation of survey scores in a course.
In addition, limitations are present in the interaction counting. For this dataset, the only interactions counted were those that took place inside the learning management system. Any interactions occurring between students and teachers outside of the learning management system are unavailable due to privacy concerns and lack of access. For some classes, this is the primary method of preferred communication and denoted as such by the instructor, leading to minimal use of the learning management system for a communication path. Some teachers also indicate that other pathways of communication result in faster response times, warning that a message through the LMS will likely be responded to in delay.
Finding/recommendations
The presence of a positive linear relationship between interactions per student and perceived quality of a course by a student shows us that increased interaction on its own can vastly improve student experiences and perception of quality. However, as mentioned above in student comments, too much interaction may have a reverse effect on the student experience. Interaction should be increased but in a meaningful way. Comments and messages that make a student feel respected, provide constructive criticism, and give credit where credit is due are the most effective path to improving student experience and perception of quality. This provides the student with a sense of belongingness. The most improvement for course evaluations will most likely be seen in those courses which the instructor makes a noticeable attempt to include and promote the students under their supervision. Those who simply increase their interactions in a course by providing non-meaningful, passive feedback will more than likely decrease their evaluation of perceived quality by students.
The action items, in this case, are not solely the courses with few interactions, but the courses with few interactions and low survey scores. These are the areas where the students are unhappy with their experience or the quality of the course. These courses are where the interaction should increase, and will thereby improve student experience as is shown above in Table 1. It is extremely important to conduct a careful examination of each college and/or course with these findings to ensure proper recommendations. If not done properly, as stated above, there could be a hindrance to the instructor’s performance scores by providing increased and unnecessary interaction.
Conclusion
One technological challenge would be to create an environment or space for instructor-learner interaction (Kolb, 2000). Activities creating interaction opportunities in an online course are part of course design, whereas the daily interaction would be part of delivery standards (Hunter, 2017).
One example for delivery standards of an online course would include meeting the Carnegie Credit Hour definition (ed.gov, 2009) Professors are provided details for a traditional face to face class regarding the credit hour, days and times of the class, and the classroom location. In an online class, the amount of time spent by the professor to meet the Carnegie Credit Hour should remain the same; however the set days and times the class run are not as clear nor concrete as a face-to-face class. The format or outline of course content, if the class is running on a Monday/Wednesday/Friday or Tuesday/Thursday schedule, is often overlooked in the development and delivery phase of an online course. This schedule does not enforce an online course being set up with a Monday/Wednesday/Friday format a student must adhere to, rather time is a guide for professors to indicate how often instructor presence or instructor interactions should take place in an online course based on the number of credit hours.
A second factor relating to online delivery is the posted office hours. Online students should be able to meet with professors using some method (asynchronously or synchronously) which adds to the interactions between the instructor and the learner. Administrators and faculty, using the findings and recommendation from this study can increase the quality of online courses providing students a sense of belonging (Baumeister, 1995) in an online learning environment.
References
Allen, I., and Seaman, J. (2015). “Grade Level: Tracking Online Education in the United States.” Babson Survey Research Group. 66 PAGE REPORT. Retrieved from http://www.onlinelearningsurvey.com/reports/gradelevel.pdf
Baker, D. (2011). Designing and orchestrating online discussions. MERLOT Journal of Online Learning and Teaching, 7/(3), 401-411. Retrieved from http://jolt.merlot.org/vol7no3/baker_0911.htm
Barkley, E., Major, C., & Cross, K. (2014). The case for collaborative learning. Collaborative Learning Techniques: A Handbook for College Faculty. Somerset, NJ: Jossey-Bass.
Bass, R. (2012). Disrupting ourselves: The problem of learning in higher education. Educause, March/April, 23-33.
Baumeister, R. F., & Leary, M. R. (1995). The need to belong: Desire for interpersonal attachments as a fundamental human motivation. Psychological Bulletin, 117(3), 497-529. http://dx.doi.org/10.1037/0033-2909.117.3.497
Bonfiglio, K., O’Bryan, A., Palavecino, P., & Willibey, H. (2016). Student Success in Online Classes: Increasing effectiveness in student-instructor interaction. Santa Clarita, CA: College of the Canyons Faculty Inquiry Group.
Brinthaupt, T. M., Fisher, L. S., Gardner, J. G., Raffo, D. M., & Woodward, J. B. (2011). What the best online teachers should do. MERLOT Journal of Online Learning and Teaching, 7(4), 515-524.
Castano-Munoz, j., Duart, J., & Vinuesa, T. (2014). The internet in face-to-face higher education: Can interactive learning improve academic achievement? British Journal of Educational Technology, 45(1), 149-159.
CHLOE. The changing landscape of online education. Quality Matters & Eduventures Survey of Chief Online Officers, 2017. Retrieved from https://www.qualitymatters.org/sites/default/files/research-docs-pdfs/CHLOE-First-Survey-Report.pdf
Cranney, M., Alexander, J. L., Wallace, L., & Alfano, L. (2011). Instructor’s discussion forum effort: Is it worth it? MERLOT Journal of Online Learning and Teaching, 7(3), 337-348. Retrieved from http://jolt.merlot.org/vol7no3/cranney_0911.pdf
ed. Gov. https://www2.ed.gov/about/offices/list/oig/aireports/x13j0003.pdf and https://www2.ed.gov/policy/highered/reg/hearulemaking/2009/credit.html ).
Ertmer, P., & Newby, T. (2013). Article update: Behaviorism, cognitivism, constructivism: Comparing critical features from an instructional design perspective. Performance Review Quarterly, 26(2), 43-71.
Findlay-Thompson, S., & Mombourquette, P. (2014). Evaluation of a flipped classroom in an undergraduate business course. Business Education & Accreditation, 6/1, 63-71.
Goldman, Z. (2011). Balancing quality and workload in asynchronous online discussions: A win-win approach for students and instructors. MERLOT Journal of Online Learning and Teaching, 7(2), 313-323. Retrieved from http://jolt.merlot.org/vol7no2/goldman_0611.pdf
Grabe, M. & Holfeld, B. (2014). Estimating the degree of failed understanding: A possible role for online technology. Journal of Computer Assisted Learning, 30, 173-186.
Hogg, N., & Lomicky, C. (2012). Connectivism in postsecondary online courses: An exploratory Factor Analysis. The Quarterly Review of Distance Education, 13(2), 95-114.
Huneycutt, T. (2013). Technology in the Classroom: The Benefits of Blended Learning. National Math + Science Initiative Blog. www. nms.org. Retrieved from http://www.nms.org/blog/TabId/58/PostId/188/technology-in-the-classroom-the-benefits-of-blended-learning.aspx
Hunter, J. (2017). Facilitation standards: A mixed methods study (Unpublished doctoral dissertation). Northcentral University, Scottsdale, Arizona.
Jaggars, S., Edgecombe, N., & Stacey, G. (2013, April). Creating an effective online instructor presence. Community College Research Center, Teachers College, Columbia University,1-7. Retrieved from http://ccrc.tc.columbia.edu/media/k2/attachments/effective-online-instructor-presence.pdf
Kearns, L. (2012). Student Assessment in Online Learning: Challenges and Effective Practices. MERLOT Journal of Online Learning and Teaching, 8(3). Retrieved from http://jolt.merlot.org/vol8no3/kearns_0912.htm
Kleinsasser, R. & Hong, Y. (2016). Online Group Work Design: Processes, Complexities, and Intricacies. TechTrends, 60(6), 569-576.
Kolb, D. (2000). 8. Learning places: Building welling thinking online. The Journal of the Philosophy of Education Society of Great Britain, 34(1), 121-133.
Kuh, G. D., & O’Donnell, K. (2013). Ensuring quality & taking high-impact practices to scale. Washington, DC: AAC&U, Association of American Colleges and Universities.
Meyer, K., & Murrell, V. (2014, Summer) A national study of theories and their important for faculty development for online training. Online Journal of Distance Learning Administration, 17/2. Retrieved 6/27/2016 from http://www.westga.edu/~distance/ojdla/summer172/Meyer_Murrell172.html
Moreillon, J. (2015, May/June). Increasing interactivity in the online learning environment: Using digital tools to support students in socially constructed meaning-making. TechTrends. 59(3), 41-47.
Poll, K., Widen, J., & Weller, S. (2014, Summer). Six instructional best practices for online engagement and retention. Journal of Online Doctorial Education, 1(1), 56-70.
Stear, S., & Mensch, S. (2012). Online learning tools for distant education. Global Education Journal, 2012(3), 57-64.
Taylor, D., & Hamdy, H. (2013). Adult learning theories: Implications for learning and teaching in medical education: AMEE Guide No. 83. Medical Teacher, 35(11), e1561-e1572. doi: 10.3109/0142159X.2013.828153.
Watts, L. (2016). Synchronous and asynchronous communication in distance learning: A review of the literature. The Quarterly Review of Distance Education, Volume 17(1), 23–32.
Weld, D., Adar, E., Chilton, L., Hoffmann, R., Horvitz, E., Koch, M., Landay, J., Lin, C., & Mausam. (2012). Personalized Online Education: A crowdsourcing challenge. Retrieved from: https://pdfs.semanticscholar.org/50fc/b0e5f921357b2ec96be9a75bfd3169e8f8da.pdf