Key Concepts in Quantitative Research
In this module, we are going to explore the nuances of quantitative research, including the main types of quantitative research, more exploration into variables (including confounding and extraneous variables), and causation.
Content includes:
- Flaws, “Proof”, and Rigor
- The Steps of Quantitative Methodology
- Major Classes of Quantitative Research
-
- Experimental versus Non-Experimental Research
- Types of Experimental Research
- Types of Non-Experimental Research
- Research Variables
- Confounding/Extraneous Variables
- Causation versus correlation/association
Objectives:
- Discuss the flaws, proof, and rigor in research.
- Describe the differences between independent variables and dependent variables.
- Describe the steps in quantitative research methodology.
- Describe experimental, quasi-experimental, and non-experimental research studies
- Describe confounding and extraneous variables.
- Differentiate cause-and-effect (causality) versus association/correlation
Flaws, Proof, and Rigor in Research
One of the biggest hurdles that students and seasoned researchers alike struggle to grasp, is that research cannot “prove” nor “disprove”. Research can only support a hypothesis with reasonable, statistically significant evidence.
Say what?
Indeed. You’ve heard it incorrectly your entire life. You will hear professors, scientists, radio ads, podcasts, and even researchers comment something to the effect of, “It has been proven that…” or “Research proves that…” or “Finally! There is proof that…”
We have been duped. Consider the “prove” word a very bad word in this course. The forbidden “P” word. Do not say it, write it, allude to it, or repeat it. And, for the love of avocados and all things fluffy, do not include the “P” word on your EBP poster. You will be deducted some major points.
We can only conclude with reasonable certainty through statistical analyses that there is a high probability that something did not happen by chance but instead happened due to the intervention that the researcher tested. Got that? We will come back to that concept but for now know that it is called “statistical significance”.
All research has flaws. We might not know what those flaws are, but we will be learning about confounding and extraneous variables later on in this module to help explain how flaws can happen.
Remember this: Sometimes, the researcher might not even know that there was a flaw that occurred. No research project is perfect. There is no 100% awesome. This is a major reason why it is so important to be able to duplicate a research project and obtain similar results. The more we can duplicate research with the same exact methodology and protocols, the more certainty we have in the results and we can start accounting for flaws that may have sneaked in.
Finally, not all research is equal. Some research is done very sloppily, and other research has a very high standard of rigor. How do we know which is which when reading an article? Well, within this module, we will start learning about some things to look for in a published research article to help determine rigor. We do not want lazy research to determine our actions as nurses, right? We want the strongest, most reliable, most valid, most rigorous research evidence possible so that we can take those results and embed them into patient care. Who wants shoddy evidence determining the actions we take with your grandmother’s heart surgery?
Independent Variables and Dependent Variables
As we were already introduced to, there are measures called “variables” in research. This will be a bit of a review but it is important to bring up again, as it is a hallmark of quantitative research. In quantitative studies, the concepts being measured are called variables (AKA: something that varies). Variables are something that can change – either by manipulation or from something causing a change. In the article snapshots that we have looked at, researchers are trying to find causes for phenomena. Does a nursing intervention cause an improvement in patient outcomes? Does the cholesterol medication cause a decrease in cholesterol level? Does smoking cause cancer?
The presumed cause is called the independent variable. The presumed effect is called the dependent variable. The dependent variable is “dependent” on something causing it to change. The dependent variable is the outcome that a researcher is trying to understand, explain, or predict.
Think back to our PICO questions. You can think of the intervention (I) as the independent variable and the outcome (O) as the dependent variable.
The independent variable is manipulated by the researcher or can be variants of influence. Whereas the dependent variable is never manipulated.
Variables do not always measure cause-and-effect. They can also measure a direction of influence.
Here is an example of that: If we compared levels of depression among men and women diagnosed with pancreatic cancer and found men to be more depressed, we cannot conclude that depression was caused by gender. However, we can note that the direction of influence clearly runs from gender to depression. It makes no sense to suggest the depression influenced their gender.
In the above example, what is the independent variable (IV) and what is the dependent variable (DV)? If you guessed gender as the IV and depression as the DV, you are correct! Important to note in this case that the researcher did not manipulate the IV, but the IV is manipulated on its own (male or female).
Researchers do not always have just one IV. In some cases, more than one IV may be measured. Take, for instance, a study that wants to measure the factors that influence one’s study habits. Independent variables of gender, sleep habits, and hours of work may be considered. Likewise, multiple DVs can be measured. For example, perhaps we want to measure weight and abdominal girth on a plant-based diet (IV).
Now, some studies do not have an intervention. We will come back to that when we talk about non-experimental research.
The point of variables is so that researchers have a very specific measurement that they seek to study.
Knowledge to application link.
Let’s look at a couple of examples:
Study |
Independent Variable(s)(Intervention/Treatment) |
Dependent Variable(s)(Effect/Results) |
Case One: An analysis of emotional intelligence in nursing leaders—focuses on the meaning of emotional intelligence specific to nurses—defines emotional intelligence, the consequences, and antecedents. A literature review is used to find information about the meaning, consequences, and antecedents of emotional intelligence. |
None – there is no intervention |
The definition of emotional intelligence. The antecedents of emotional intelligence. |
Case Two: In this study, nurses use protocol hand hygiene for their own hands and patient hands to examine if the hand hygiene protocol will decrease hospital-acquired infections in the Intensive Care Unit. |
Hand hygiene for nurses and patients. Nurse in-service training on hand hygiene for nurses and patients. |
Hospital-acquired infection rates in the ICU. |
Now you try! Identify the IVs and DVs:
Study |
Independent Variable(s)(Intervention/Treatment) |
Dependent Variable(s)(Effect/Results) |
Case Three: A nurse wants to know if extra education about healthy lifestyles with a focus on increasing physical activity with adolescents will increase their physical activity levels and impact their heart rates and blood pressures over a 6-month time. Data is collected before intervention and after intervention at multiple intervals. A control group and intervention group is used. Randomized assignment to groups is used. (True Experimental design with intervention group, control group, and randomization.) |
|
|
Case Four: Playing classical music for college students was examined to study if it impacts their grades—music was played for college students in the study and their post music grades were compared to their pre-music grades. |
|
|
Case Five: A nurse researcher studies the lived experiences of registered nurses in their first year of nursing practice through a one-on-one interview. The nurse researcher records all the data and then has it transcribes to analysis themes that emerge from the 28 nurses interviewed. |
|
|
IV and DV Case Studies (Leibold, 2020)
Well done!
Answers:
Case Three: Independent variable: Healthy Lifestyle education with a focus on physical activity; Dependent variable: Physical activity rate before and after education intervention, Heart rate before and after education intervention, Blood pressures before and after education intervention.
Case Four: Independent variable: Playing classical music; Dependent variable: Grade point averages post classical music, compared to pre-classical music.
Case Five: Independent variable: No independent variable as there is no intervention. Dependent variable: The themes that emerge from the qualitative data.
The Steps in Quantitative Research Methodology
Now, as we learned in the last module, quantitative research is completely objective. There is no subjectivity to it. Why is this? Well, as we have learned, the purpose of quantitative research is to make an inference about the results in order to generalize these results to the population.
In quantitative studies, there is a very systematic approach that moves from the beginning point of the study (writing a research question) to the end point (obtaining an answer). This is a very linear and purposeful flow across the study, and all quantitative research should follow the same sequence.
- Identifying a problem and formulating a research question. Quantitative research begins with a theory. As in, “something is wrong and we want to fix it or improve it”. Think back to when we discussed research problems and formulating a research question. Here we are! That is the first step in formulating a quantitative research plan.
- Formulate a hypothesis. This step is key. Researchers need to know exactly what they are testing so that testing the hypothesis can be achieved through specific statistical analyses.
- A thorough literature review. At this step, researchers strive to understand what is already known about a topic and what evidence already exists.
- Identifying a framework. When an appropriate framework is identified, the findings of a study may have broader significance and utility (Polit & Beck, 2021).
- Choosing a study design. The research design will determine exactly how the researcher will obtain the answers to the research question(s). The entire design needs to be structured and controlled, with the overarching goal of minimizing bias and errors. The design determines what data will be collected and how, how often data will be collected, what types of comparisons will be made. You can think of the study design as the architectural backbone of the entire study.
- Sampling. The researcher needs to determine a subset of the population that is to be studied. We will come back to the sampling concept in the next module. However, the goal of sampling is to choose a subset of the population that adequate reflects the population of interest.
- Instruments to be used to collect data (with reliability and validity as a priority). Researchers must find a way to measure the research variables (intervention and outcome) accurately. The task of measuring is complex and challenging, as data needs to be collected reliably (measuring consistently each time) and valid. Reliability and validity are both about how well a method measures something. The next module will cover this in detail.
- Obtaining approval for ethical/legal human rights procedures. As we will learn in an upcoming module, there needs to be methods in place to safeguard human rights.
- Data collection. The fun part! Finally, after everything has been organized and planned, the researcher(s) begin to collect data. The pre-established plan (methodology) determines when data collection begins, how to accomplish it, how data collection staff will be trained, and how data will be recorded.
- Data analysis. Here comes the statistical analyses. The next module will dive into this.
- Discussion. After all the analyses have been complete, the researcher then needs to interpret the results and examine the implications. Researchers attempt to explain the findings in light of the theoretical framework, prior evidence, theory, clinical experience, and any limitations in the study now that it has been completed. Often, the researcher discusses not just the statistical significance, but also the clinical significance, as it is common to have one without the other.
- Summary/references. Part of the final steps of any research project is to disseminate (AKA: share) the findings. This may be in a published article, conference, poster session, etc. The point of this step is to communicate to others the information found through the study. All references are collected so that the researchers can give credit to others.
- Budget and funding. As a last mention in the overall steps, budget and funding for research is a consideration. Research can be expensive. Often, researchers can obtain a grant or other funding to help offset the costs.
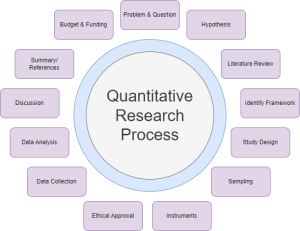
Edit: Steps in Quantitative Research video. Step 12 should say “Dissemination” (sharing the results).
Experimental, Quasi-Experimental, and Non-Experimental Studies
To start this section, please watch this wonderful video by Jenny Barrow, MSN, RN, CNE, that explains experimental versus nonexperimental research.
(Jenny Barrow, 2019)
Now that you have that overview, continue reading this module.
Experimental Research: In experimental research, the researcher is seeking to draw a conclusion between an independent variable and a dependent variable. This design attempts to establish cause-effect relationships among the variables. You could think of experimental research as experimenting with “something” to see if it caused “something else”.
A true experiment is called a Randomized Controlled Trial (or RCT). An RCT is at the top of the echelon as far as quantitative experimental research. It’s the gold standard of scientific research. An RCT, a true experimental design, must have 3 features:
- An intervention: The experiment does something to the participants by the option of manipulating the independent variable.
- Control: Some participants in the study receive either the standard care, or no intervention at all. This is also called the counterfactual – meaning, it shows what would happen if no intervention was introduced.
- Randomization: Randomization happens when the researcher makes sure that it is completely random who receives the intervention and who receives the control. The purpose is to make the groups equal regarding all other factors except receipt of the intervention.
Note: There is a lot of confusion with students (and even some researchers!) when they refer to “random assignment” versus “random sampling”. Random assignment is a signature of a true experiment. This means that if participants are not truly randomly assigned to intervention groups, then it is not a true experiment. We will talk more about random sampling in the next module.
One very common method for RCT’s is called a pretest-posttest design. This is when the researcher measures the outcome before and after the intervention. For example, if the researcher had an IV (intervention/treatment) of a pain medication, the DV (pain) would be measured before the intervention is given and after it is given. The control group may just receive a placebo. This design permits the researcher to see if the change in pain was caused by the pain medication because only some people received it (Polit & Beck, 2021).
Another experimental design is called a crossover design. This type of design involves exposing participants to more than one treatment. For example, subject 1 first receives treatment A, then treatment B, then treatment C. Subject 2 might first receive treatment B, then treatment A, and then treatment C. In this type of study, the three conditions for an experiment are met: Intervention, randomization, and control – with the subjects serving as their own control group.
Control group conditions can be done in 4 ways:
- No intervention is used; control group gets no treatment at all
- “Usual care” or standard of care or normal procedures used
- An alternative intervention is uses (e.g. auditory versus visual stimulation)
- A placebo or pseudo-intervention, presumed to have no therapeutic value, is used
Quasi-Experimental Research: Quasi-experiments involve an experiment just like true experimental research. However, they lack randomization and some even lack a control group. Therefore, there is implementation and testing of an intervention, but there is an absence of randomization.
For example, perhaps we wanted to measure the effect of yoga for nursing students. The IV (intervention of yoga) is being offered to all nursing students and therefore randomization is not possible. For comparison, we could measure quality of life data on nursing students at a different university. Data is collected from both groups at baseline and then again after the yoga classes. Note, that in quasi-experiments, the phrase “comparison group” is sometimes used instead of “control group” against which outcome measures are collected.
Sometimes there is no comparison group either. This would be called a one-group pretest-posttest design.
Non-Experimental Research: Sometimes, cause-problem research questions cannot be answered with an experimental or quasi-experimental design because the IV cannot be manipulated. For example, if we want to measure what impact prerequisite grades have on student success in nursing programs, we obviously cannot manipulate the prerequisite grades. In another example, if we wanted to investigate how low birth weight impacts developmental progression in children, we cannot manipulate the birth weight. Often, you will see the word “observational” in lieu of non-experimental researcher. This does not mean the researcher is just standing and watching people, but instead it refers to the method of observing data that has already been established without manipulation.
There are various types of non-experimental research:
Correlational research: A correlational research design investigates relationships between two variables (or more) without the researcher controlling or manipulating any of them. In the example of prerequisites and nursing program success, that is a correlational design. Consider hypothetically, a researcher is studying a correlation between cancer and marriage. In this study, there are two variables: disease and marriage. Let us say marriage has a negative association with cancer. This means that married people are less likely to develop cancer.
Cohort design (also called a prospective design): In a cohort study, the participants do not have the outcome of interest to begin with. They are selected based on the exposure status of the individual. They are then followed over time to evaluate for the occurrence of the outcome of interest. Cohorts may be divided into exposure categories once baseline measurements of a defined population are made. For example, the Framingham Cardiovascular Disease Study (CVD) used baseline measurements to divide the population into categories of CVD risk factors. Another example: An example of a cohort study is comparing the test scores of one group of people who underwent extensive tutoring and a special curriculum and those who did not receive any extra help. The group could be studied for years to assess whether their scores improve over time and at what rate.
Retrospective design: In retrospective studies, the outcome of interest has already occurred (or not occurred – e.g., in controls) in each individual by the time s/he is enrolled, and the data are collected either from records or by asking participants to recall exposures. There is no follow-up of participants. For example, a researcher might examine the medical histories of 1000 elderly women to identify the causes of health problems.
Case-control design: A study that compares two groups of people: those with the disease or condition under study (cases) and a very similar group of people who do not have the condition. For example, investigators conducted a case-control study to determine if there is an association between colon cancer and a high fat diet. Cases were all confirmed colon cancer cases in North Carolina in 2010. Controls were a sample of North Carolina residents without colon cancer.
Descriptive research: Descriptive research design is a type of research design that aims to obtain information to systematically describe a phenomenon, situation, or population. More specifically, it helps answer the what, when, where, and how questions regarding the research problem, rather than the why. For example, the researcher might wish to discover the percentage of motorists who tailgate – the prevalence of a certain behavior.
There are two other designs to mention, which are both on a time continuum basis.
Cross-sectional design: All data are collected at a single point in time. Retrospective studies are usually cross-sectional. The IV usually concerns events or behaviors occurring in the past. One cross-sectional study example in medicine is a data collection of smoking habits and lung cancer incidence in a given population. A cross-sectional study like this cannot solely determine that smoking habits cause lung cancer, but it can suggest a relationship that merits further investigation. Cross-sectional studies serve many purposes, and the cross-sectional design is the most relevant design when assessing the prevalence of disease, attitudes and knowledge among patients and health personnel, in validation studies comparing, for example, different measurement instruments, and in reliability studies.
Longitudinal design: Data are collected two or more times over an extended period. Longitudinal designs are better at showing patterns of change and at clarifying whether a cause occurred before an effect (outcome). A challenge in longitudinal studies is attrition or the loss of participants over time. In a longitudinal study subjects are followed over time with continuous or repeated monitoring of risk factors or health outcomes, or both. Such investigations vary enormously in their size and complexity. At one extreme a large population may be studied over decades. An example of a longitudinal design is a multiyear comparative study of the same children in an urban and a suburban school to record their cognitive development in depth.
Confounding and Extraneous Variables
Confounding variables are a type of extraneous variable that occur which interfere with or influence the relationship between the independent and dependent variables. In research that investigates a potential cause-and-effect relationship, a confounding variable is an unmeasured third variable that influences both the supposed cause and the supposed effect.
It’s important to consider potential confounding variables and account for them in research designs to ensure results are valid. You can imagine that if something sneaks in to influence the measured variables, it can really muck up the study!
Here is an example:
You collect data on sunburns and ice cream consumption. You find that higher ice cream consumption is associated with a higher probability of sunburn. Does that mean ice cream consumption causes sunburn?
Here, the confounding variable is temperature: hot temperatures cause people to both eat more ice cream and spend more time outdoors under the sun, resulting in more sunburns.
Above: Conceptual Model of Confounding Variables
To ensure the internal validity of research, the researcher must account for confounding variables. If he/she fails to do so, the results may not reflect the actual relationship between the variables that they are interested in.
For instance, they may find a cause-and-effect relationship that does not actually exist, because the effect they measure is caused by the confounding variable (and not by the independent variable).
Here is another example:
The researcher finds that babies born to mothers who smoked during their pregnancies weigh significantly less than those born to non-smoking mothers. However, if the researcher does not account for the fact that smokers are more likely to engage in other unhealthy behaviors, such as drinking or eating less healthy foods, then he/she might overestimate the relationship between smoking and low birth weight.
Extraneous variables are any variables that the researcher is not investigating that can potentially affect the outcomes of the research study. If left uncontrolled, extraneous variables can lead to inaccurate conclusions about the relationship between IVs and DVs.
Extraneous variables can threaten the internal validity of a study by providing alternative explanations for the results. In an experiment, the researcher manipulates an independent variable to study its effects on a dependent variable.
Here is an example:
In a study on mental performance, the researcher tests whether wearing a white lab coat, the independent variable (IV), improves scientific reasoning, the dependent variable (DV).
Students from a university are recruited to participate in the study. The researcher manipulates the independent variable by splitting participants into two groups:
- Participants in the experimental group are asked to wear a lab coat during the study.
- Participants in the control group are asked to wear a casual coat during the study.
All participants are given a scientific knowledge quiz, and their scores are compared between groups.
When extraneous variables are uncontrolled, it’s hard to determine the exact effects of the independent variable on the dependent variable, because the effects of extraneous variables may mask them.
Uncontrolled extraneous variables can also make it seem as though there is a true effect of the independent variable in an experiment when there’s actually none.
In the above experiment example, these extraneous variables can affect the science knowledge scores:
- Participant’s major (e.g., STEM or humanities)
- Participant’s interest in science
- Demographic variables such as gender or educational background
- Time of day of testing
- Experiment environment or setting
If these variables systematically differ between the groups, you can’t be sure whether your results come from your independent variable manipulation or from the extraneous variables.
In summary, an extraneous variable is anything that could influence the dependent variable. A confounding variable influences the dependent variable, and also correlates with or causally affects the independent variable.
Above: Extraneous and Confounding Variables
Cause-and-Effect (Causality) Versus Association/Correlation
A very important concept to understand is cause-and-effect, also known as causality, versus correlation. Let’s look at these two concepts in very simplified statements. Causation means that one thing caused another thing to happen. Correlation means there is some association between the two thing we are measuring.
It would be nice if it were as simple as that. These two concepts can indeed by confused by many. Let’s dive deeper.
Two or more variables are considered to be related or associated, in a statistical context, if their values change so that as the value of one variable increases or decreases so does the value of the other variable (or the opposite direction).
For example, for the two variables of “hours worked” and “income earned”, there is a relationship between the two if the increase in hours is associated with an increase in income earned.
However, correlation is a statistical measure that describes the size and direction of a relationship between two or more variables. A correlation does not automatically mean that the change in one variable caused the change in value in the other variable.
Theoretically, the difference between the two types of relationships is easy to identify — an action or occurrence can cause another (e.g. smoking causes an increase in the risk of developing lung cancer), or it can correlate with another (e.g. smoking is correlated with alcoholism, but it does not cause alcoholism). In practice, however, it remains difficult to clearly establish cause and effect, compared with establishing correlation.
Simplified in this image, we can say that hot and sunny weather causes an increase in ice cream consumption. Similarly, we can demise that hot and sunny weather increases the incidence of sunburns. However, we cannot say that ice cream caused a sunburn (or that a sunburn increases consumption of ice cream). It is purely coincidental. In this example, it is pretty easy to anecdotally surmise correlation versus causation. However, in research, we have statistical tests that help researchers differentiate via specialized analyses.
Here is a great Khan Academy video of about 5 minutes that shows a worked example of correlation versus causation with regard to sledding accidents and frostbite cases:
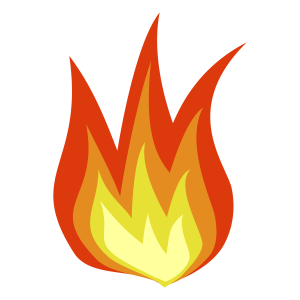
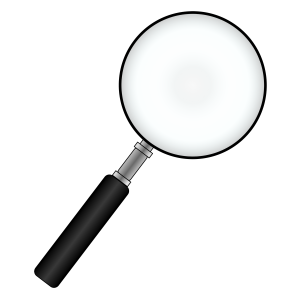
References & Attribution
“Light bulb doodle” by rawpixel licensed CC0.
“Magnifying glass” by rawpixel licensed CC0
“Orange flame” by rawpixel licensed CC0.
Jenny Barrow. (2019). Experimental versus nonexperimental research. https://www.youtube.com/watch?v=FJo8xyXHAlE
Leibold, N. (2020). Research variables. Measures and Concepts Commonly Encountered in EBP. Creative Commons License: BY NC
Polit, D. & Beck, C. (2021). Lippincott CoursePoint Enhanced for Polit’s Essentials of Nursing Research (10th ed.). Wolters Kluwer Health.